What is Data Quality: Understanding Its Impact on Business Intelligence
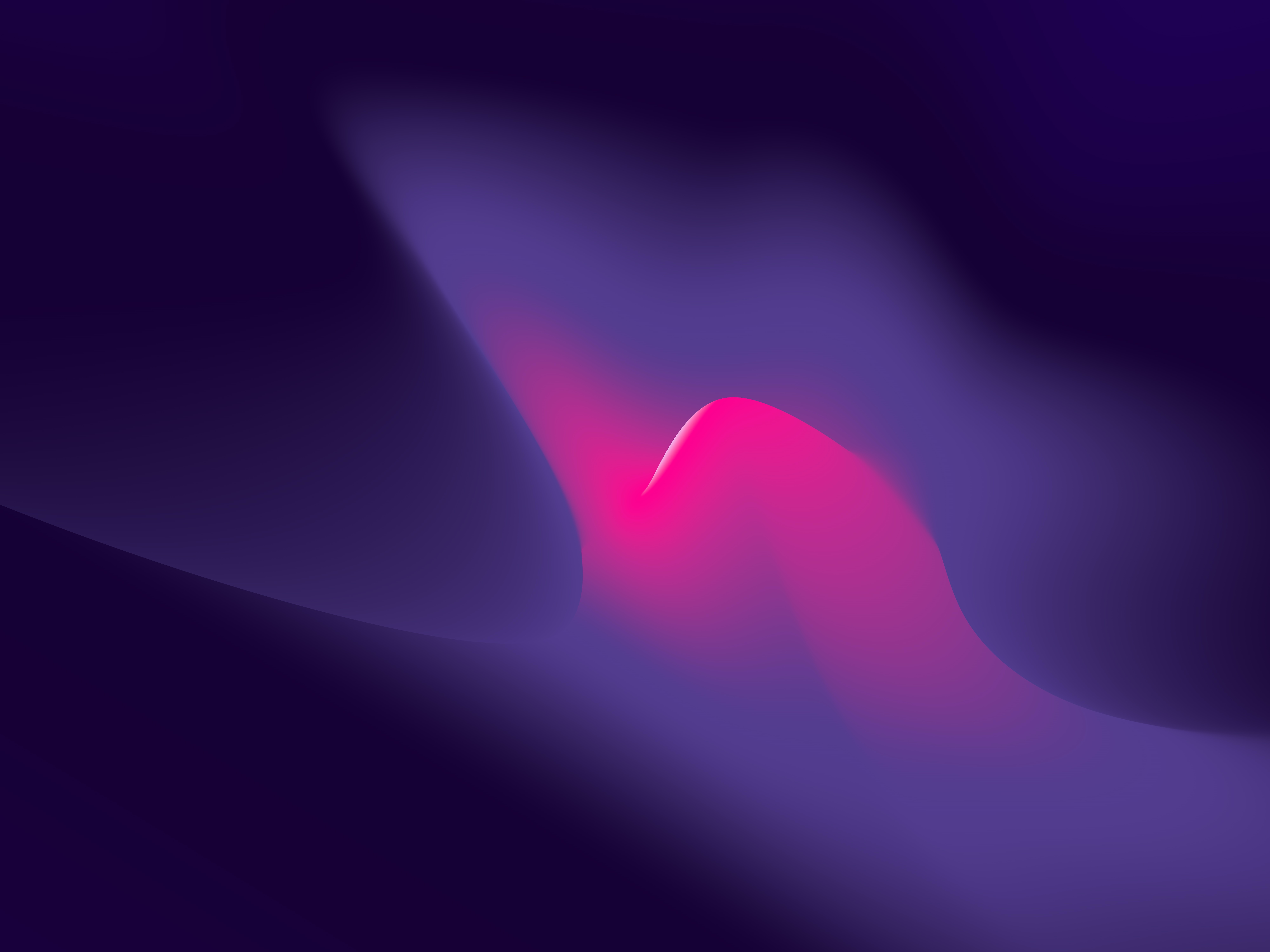
Defining Data Quality
When we converse about "Data Quality," it's integral to comprehend that this is an umbrella term that accommodates various facets and dimensions. Data quality is founded on multiple dimensions; each bearing its own weight on the overall quality our business intelligence derives. Whether it's the accuracy, the completeness, or the consistency of data, each dimension plays a pivotal role in determining the value and relevance of the information at our disposal.
Accuracy of data refers to the proximity of data value to its true or original form. Simply put, it is about the data correctly representing the real-world instances they're intended to capture. Wrong entries or transcription errors can cause inaccuracies and potentially lead to false insights.
On the other hand, completeness of data indicates that the necessary entries in the data set are present and not missing. This is crucial as missing data may lead to skewed interpretations and flawed analysis.
Consistency measures the uniformity of data across different data sources. It looks at whether the same data point corresponds to the same reality, irrespective of where and when it was recorded. Inconsistent data can yield conflicting trends and findings, thereby obstructing the formulation of a sound business strategy.
Other dimensions such as relevance, timeliness, and usability, too, shape the quality of data. Every dimension contributes uniquely. Thus, a holistic approach is needed while assessing data quality.
Importance of High-Quality Data
The significance of high-quality data often gets underscored, while its implications run wide and far-reaching. High-quality data serves as the backbone for strategic business decisions, opening new vistas of opportunities, and driving innovation. This becomes crucial in the age of digital transformation, where data-driven practices have the power to shift the competitive landscape in favor of those who harness this potential best.
Inferior data quality, on the contrary, poses potential risks, paving the way for incorrect decisions, missed opportunities, and spiraling costs incurred due to data rectification. In fact, a Gartner study reveals that poor data quality can rack up costs amounting to an average $15 million per year. This statistic alone emphasizes how low data quality could be detrimental to a business’s financial health.
High-quality data fortifies trust. It forms a robust foundation for leveraging machine learning and AI, and helps create an accurate understanding of the market and customer base. Moreover, it can streamline operations, improve customer satisfaction, and culminate in a competitive advantage. Hence, prioritizing data quality isn't just a good habit – in a world increasinly reliant on data insights, it's a business imperative. Stakes couldn't be higher, underscoring a pressing need for businesses to invest in data quality management and improvement.
Therefore, it becomes apparent that accurate, complete, and consistent data holds the power to optimize business performance, propel strategic decision-making, and unlock new growth avenues. At the same time, it stresses that poor data quality can significantly impede these goals.
Data Quality and Machine Learning
High-quality data isn't just needed for traditional business intelligence but has a far-reaching impact on newer technology applications such as Machine Learning (ML) and Artificial Intelligence (AI). Ensuring data quality in these contexts marks the difference between successful models and failed predictions.
One notably salient aspect of machine learning is its reliance on data for training. Machine learning algorithms learn from data, extract meaningful insights, make predictions, and drive decisions. If the quality of this data is compromised, the model's predictions can't be trusted.
For instance, inaccurate data can lead machine learning models to form incorrect associations, therefore birthing erroneous predictions. Similarly, incomplete data may lead to biased predictions, as the model hasn't learned from the full spectrum of possibilities. Data inconsistency could confuse a model, teaching it incorrect or conflicting rules. In essence, poor quality data breeds poor quality outcomes.
Equally important, clean, high-quality data is key to AI transparency and interpretability. Given that AI algorithms are often deemed as "black boxes," strong data quality can provide a clearer understanding of how given inputs generate specific outputs, improving overall AI transparency.
Impact of Data Quality on Business Intelligence
In the sphere of business intelligence, the adage "Garbage in, garbage out," holds true. Leveraging BI tools to the fullest hinges largely on the quality of data that feeds into them.
Business Intelligence utilizes data to extract valuable insights and facilitate strategic decision-making. If the data at the disposal of a BI tool is inaccurate, incomplete, or inconsistent, the insights generated will likely misrepresent reality, leading businesses down faulty paths.
Moreover, BI is about presenting the most relevant findings to the right people at the right time. However, this relevance is compromised if the data doesn't aptly reflect the situation or scenario it aims to depict. Consequently, decision-makers would be faced with inaccurate or irrelevant information, clouding their abilities to make effective, informed decisions.
Inadequate data quality can also lead to inefficiencies in the usage of BI tools. Consider the time and resources spent on troubleshooting errors, reconciling inconsistencies, and fixing inaccurate information—instead of utilizing insights to drive business growth.
These examples highlight an irrefutable truth: the insights drawn from business intelligence are profoundly influenced by the quality of data driving analytics. A commitment to ensuring data quality aids in maximizing returns on your business intelligence investment, facilitating informed, strategic decision-making that aligns with your overarching business objectives.
Approaches to Improve Data Quality
The pathway towards improved data quality isn't overly complicated, but it requires deliberate efforts, strategies, and best practices to ensure data is constantly polished and cleaned for optimal utilization.
Start with a robust data governance policy, clearly defining who is responsible for what data, how data is collected, stored, and accessed, and what steps are necessary in case of discrepancies. This overarching guidance provides employees a clear roadmap and helps uphold data quality standards throughout the organization.
Investing in data validation tools comes next. From preventing duplicate entries to detecting anomalies or inconsistencies, validation tools play a crucial role in maintaining data accuracy and consistency.
Data cleaning, though it may seem a daunting chore, is an irreplaceable step. Fixing errors, handling missing entries, and correcting inconsistencies are some of the tasks involved. Automation, use of machine learning tools, and data imputation techniques can make this process more efficient and less resource-intensive.
Ensure an ongoing data quality assessment. Regular audits help detect slipping standards, emerging problems, and areas of improvement, thus facilitating a consistent and standardized level of high-quality data.
Case Study: High-Quality Data in Action
To illustrate how beneficial high-quality data can be, let's explore a real-world example. A multinational conglomerate faced challenges with their sales forecasting due to inaccurate, incomplete, and inconsistent data. The lack of high-quality data was leading to incorrect predictions, which was affecting their inventory management and, subsequently, the customer experience.
Identifying the root cause, the company decided to invest in data quality improvement strategies. They undertook a comprehensive data cleaning operation, engaged data validation tools, and set up stringent data governance policies. The company also trained their workforce on these policies, ensuring that everyone understood the importance of maintaining data integrity.
The results? The company was soon able to generate more accurate sales forecasts. High-quality data not only improved the prediction capabilities of their models but also helped them understand market trends better. Consequently, they could manage their inventory more effectively, which led to enhanced customer satisfaction and increased sales.
What this case underlines is the transformative power of prioritizing and investing in data quality. It demonstrates that data quality is a critical driving force capable of optimizing business performance and fueling growth strategies.
Role of Data Quality in Regulated Industries
When it comes to regulated industries like financial services, healthcare, and government bodies, the importance of data quality becomes even more prominent. Regulations not only mandate data accuracy but also demand proper documentation, thus making data quality a center-stage player.
In banking and financial services, wrong data could lead to significant calculations errors, potentially causing financial losses or non-compliance penalties. With healthcare, incorrect information could have grave impacts on patient outcomes. In government organizations, data errors and inconsistencies can lead to decision-making problems at various levels, ultimately affecting public services.
These industries have to navigate through both the challenges of maintaining high-quality data and demonstrating adherence to data regulations. Successfully doing so not only upholds compliance but also fuels more accurate predictions, better risk management, improved customer experiences, and enhanced operational efficiencies.
Looking Ahead: Data Quality in the Age of Big Data and AI
As we delve into the era of Big Data and AI, the significance of data quality is intensified. Proliferating data volumes and increased complexity make it more challenging to maintain data quality. Yet, it's this data that feeds our AI models, powering forward-looking predictions and ground-breaking discoveries.
Enterprises, therefore, need to overhaul their approach towards data management. Building a culture that values data quality, investing in advanced data management tools, training employees, and ensuring top-down commitment are some of the steps to consider as we move into the future of data-centric businesses.
As technologies evolve rapidly, the ability to harness quality data will delineate industry frontrunners from the rest. It necessitates not just the right technology investments, but also organizational changes that put data quality at the forefront.
Amidst all this, one cannot stress enough - data quality is no longer optional. It's a business essential, a key differentiator, and a pivotal factor that can shape the future trajectory of enterprises as they navigate the brave new world of AI and big data. It is the insight-driven path leading enterprises to the crest of their respective industries. Embracing this reality ensures a future where data-powered decisions drive growth and success.