Data Quality: Key to Reliable Business Intelligence
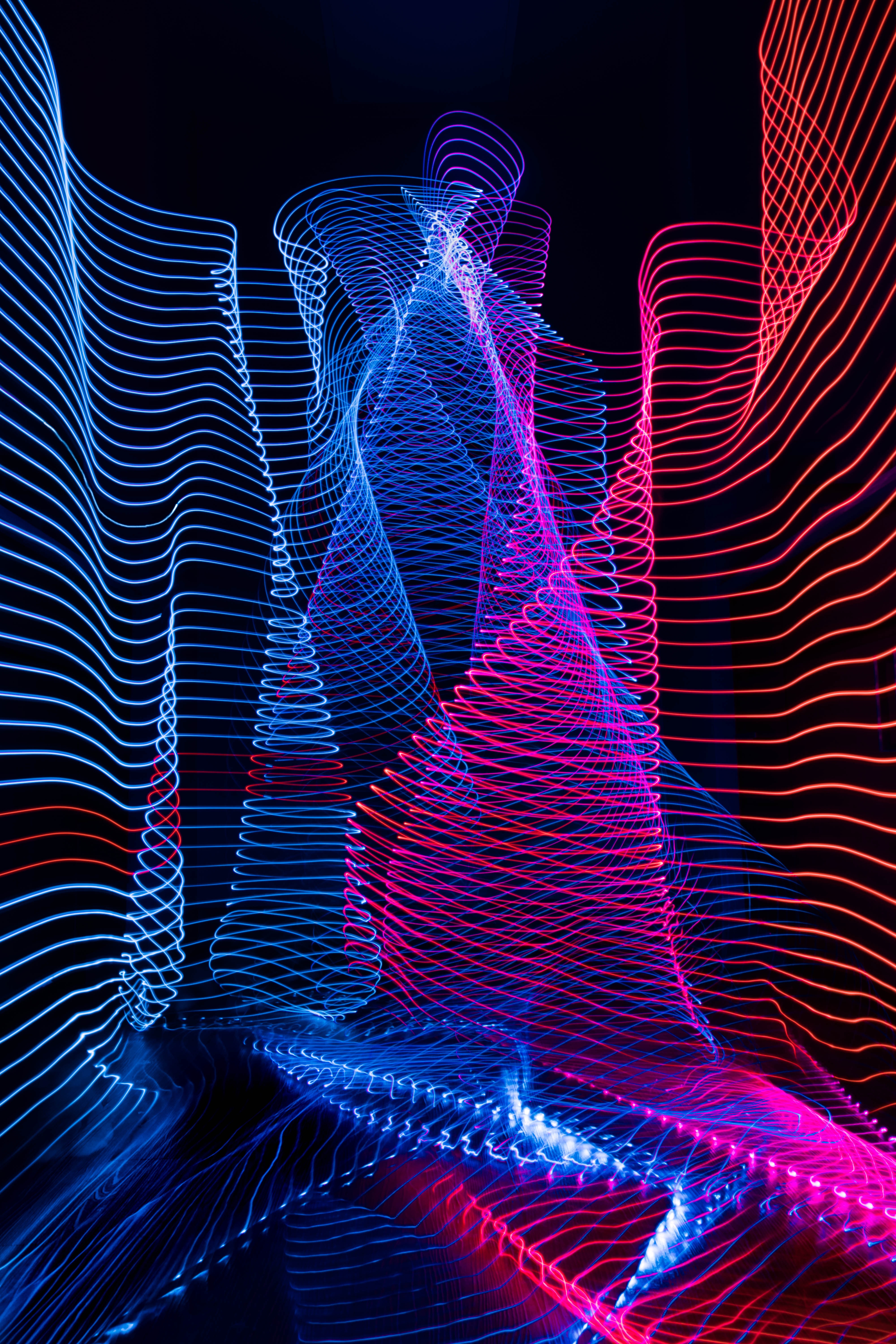
Data Quality: Breaking Down the Concept
Data quality, at its core, refers to the degree of excellence exhibited by the data in fulfilling the intended usage. When we explore the deeper layers of data quality, it unfolds into various dimensions – accuracy, completeness, consistency, relevance, and timeliness form the key pillars.
Accuracy is about how well the data reflects the real-world scenario it represents. Accurate data is free from errors and falsehoods, making it a reliable source of information. Completeness, on the other hand, is about having no omissions – every required data point is included and available.
Consistency refers to the uniformity in data across various data sets and databases. It means the same piece of information maintains its state and representation no matter where in the system it's referenced. Relevance talks about the pertinence of data to the task at hand. Relevant data is that which is suitable and applicable for the business operations or decision-making process.
Lastly, timeliness refers to the availability of data exactly when it is needed. Timely data is up-to-date, ensuring the decisions made based on it are in line with the current market trends or business state.
Together, these facets paint a clear picture of data quality. A holistic approach towards maintaining data quality involves constant tending to each of these aspects. In this era, where data is the bloodline of businesses, the significance of data quality is paramount. From daily operations to strategic decision-making, data quality plays an undeniable role in the efficient functioning and success of modern businesses. Good data quality is not just desirable – it’s the lifeblood for any organization wishing to extract meaningful and useful insights from its data.
Why Is Data Quality Important?
In a world awash with data, the importance of data quality can't be overstated. It acts as a backbone for various critical business aspects, including decision-making, operational efficiency, customer satisfaction, and compliance.
Within the realm of decision-making, high-quality data is the compass that guides businesses toward the right choices. Whether it's understanding customer preferences, evaluating the performance of marketing strategies, or making investment decisions, such insights rely heavily on the availability of precise and reliable data.
From an operational perspective, data quality plays a significant role in enhancing efficiency and productivity. When the data is accurate, timely, and relevant, teams can carry out their tasks more effectively, reducing errors and boosting productivity.
Data quality also has profound implications for customer satisfaction. Accurate data can offer valuable insights into customer behavior and preferences, helping businesses fine-tune their services and deliver a more personalized and satisfying customer experience.
In today's regulatory landscape, poor data quality can lead to non-compliance with data protection laws, resulting in hefty fines and reputational damage. High-quality data ensures better regulatory compliance, reducing the risk of compliance failures and their related costs.
The Significance of Data Quality in Business Intelligence
Business Intelligence (BI) stands at the intersection of data and decision-making. In this context, data quality elevates from being important to being indispensable. Drawing reliable and action-oriented insights from BI tools requires a solid foundation of high-quality data.
Firstly, accurate data is crucial for the generation of precise analytic reports which form the basis for strategic planning and decision-making. A single point of inaccuracy could set off a chain reaction, leading to faulty analysis and incorrect decisions. Keeping accuracy at the core of data quality ensures the BI insights are dependable.
Completeness of data is another significant factor. BI tools require comprehensive data to churn out meaningful analytics. Incomplete data can produce skewed results that could mislead decision makers. Therefore, ensuring data completeness can augment the reliability of BI outcomes.
Moreover, consistency in data is of paramount importance in BI. With many businesses leveraging multiple data sources, ensuring uniformity in data representation becomes crucial. Without consistent data, creating comparative reports or conducting trend analysis can be a real challenge.
The relevance of data contributes significantly to the efficiency of BI processes. Sifting through troves of irrelevant data wastes precious time and computational power. A focus on data relevance ensures the BI tools work on data that matters, producing valuable insights faster.
Finally, the importance of timeliness of data in the realm of BI cannot be overstated. BI is about providing insights for immediate decision-making. Outdated data can lead to decisions that are out of sync with current trends or market conditions.
How to Measure Data Quality
Measuring data quality is an essential step to better manage it. By setting up appropriate metrics, businesses can quantify the current standard of their data quality, enabling them to better aim their improvement efforts. Let's delve into several key dimensions one can consider while setting up data quality metrics.
The accuracy of data can be measured by checking a sample of the data against a reliable source or standard. By identifying discrepancies or errors, the accuracy rate can be calculated, providing a clear metric for the current state of data accuracy.
Data completeness involves examining the data to check if any essential fields are left empty or incomplete. Calculating the percentage of complete records out of the total number of records gives a quantifiable measure of data completeness.
Consistency in data can be measured by verifying that standardized formats, definitions, and rules are followed across all datasets. The proportion of data entries that abide by these standards gives you the consistency rate.
For measuring the relevance of data, it's important to look at how well the data aligns with the current business requirements and objectives. Businesses can define specific measures based on how often the data is utilized, the value it provides, or how closely it matches with the required data criteria.
The timeliness of data can be measured by assessing how up-to-date and current the data is compared to a certain standard or requirement. This could be done by figuring out the average age of the data or identifying the number of outdated records.
Measuring data quality can be a challenging task, given its many dimensions and intricacies, but it's a vital one. As the old adage goes, "You can't manage what you can't measure." Only by quantifying data quality can businesses hope to manage and improve it to harness their data's true potential. With a better understanding and measurement of their data quality, businesses can make strategic decisions, improve their operations, and drive business growth.
Steps to Improve Data Quality
Improving data quality is not just a task – it's an ongoing, strategic, and structured process that permeates the entire data lifecycle. Let's explore the steps involved in this meticulous process.
Firstly, establishing data standards lays a strong foundation for high data quality. It includes predefined formats, definitions, and guidelines that standardize how data is collected and stored, fostering consistency and accuracy.
The next step is to leverage technology to automate data quality checks. This can include implementing data validation rules, using machine learning algorithms to detect anomalies, or integrating data quality tools that can automate the process of data cleansing.
Data profiling also plays a pivotal role in enhancing data quality. By examining the data in detail, businesses can identify trends, patterns, inconsistencies, abnormalities, and redundancies that can be rectified. It allows for an in-depth understanding of the current state of data quality, enabling effective improvement measures.
Data cleansing is a vital step in the journey to improve data quality. This involves identifying and rectifying errors, filling missing values, eliminating duplicates, and updating outdated records. By purging the data of inaccuracies and inconsistencies, businesses can improve the quality and reliability of their data.
Establishing a robust data governance framework is essential to maintaining and improving data quality in the long term. This includes defining clear roles and responsibilities for data quality management, establishing ongoing data quality audits, and fostering a culture that values high-quality data.
Last but not least, is the continuous monitoring and measurement of data quality. By setting up relevant data quality metrics and regularly tracking them, businesses can ensure they maintain a high level of data quality. This provides ongoing insights into their data quality efforts and allows for continuous improvements.
By walking through these steps, businesses can significantly uplift their data quality. Keeping in mind that the road to data quality is not a one-time journey, but an ongoing commitment, businesses can ensure that the investment of time, resources, and efforts into improving data quality yields valuable, long-term dividends. High-quality data, after all, is the cornerstone of successful business intelligence, making it an investment worth making.
Challenges in Ensuring Data Quality
While the benefits of high-quality data are compelling, the journey to achieving data quality is fraught with obstacles. Overcoming these challenges requires foresight, strategic planning, and a relentless commitment to improvement.
One prevalent challenge is the sheer volume and complexity of data. Managing copious amounts of data from various sources, each with its unique formats and standards, can be a daunting task. Utilizing advanced data integration and data quality tools can help address this issue, automating the processes of checking, cleaning, and validating data.
Data inaccuracies pose another significant challenge. These inaccuracies can creep in during data entry or data integration processes and can significantly impact the reliability of the data. Implementing stringent data validation rules and regular auditing can help prevent and correct data inaccuracies, enhancing data accuracy, and reliability.
Achieving data consistency is a challenge, particularly in large organizations with numerous data sources. Different departments or data sources might use different formats, definitions, and standards, leading to inconsistencies. Establishing enterprise-wide data governance that ensures all data adheres to a single set of standards can help achieve data consistency.
Keeping data up-to-date, another challenge, grows trickier in the age of real-time data. Outdated data limits its utility for decision-making and strategic planning. Implementing real-time data governance and regularly updating databases can help maintain the timeliness and relevance of data.
Case Study: Improved Business Intelligence through Enhanced Data Quality
For a concrete understanding of the impact of data quality on business intelligence, consider a real-life example: a leading e-commerce giant grappling with an exponentially growing user base and an extensive product range.
Within this complex structure, the company found itself entrenched in intricate data – user behavior, product insights, logs, clicks, purchases, and reviews – a treasure trove of data, but one that posed challenges in terms of data management and quality.
Recognizing the potential of the data, the company decided to invest seriously in enhancing its data quality. They implemented a data governance strategy focusing on improving the precision, completeness, consistency, and timeliness of their data.
Next, a robust data quality tool was introduced into their system that helped streamline the data cleansing and validation processes. This tool automated various tasks such as de-duplication, standardization, validation, and enrichment of data.
Moreover, they set up a continuous monitoring system, using automated checks and alerts to detect irregularities or deviations from established data quality thresholds. This proactive approach helped maintain the high quality of data consistently over time.
The improvement in data quality radically transformed their business intelligence outcomes. The accurate, consistent, and timely data provided precise insights into user behavior, enabling the company to personalize their product recommendations to each user. Comprehensive product logs and real-time availability status prevented any stock-out situations, improving the customer experience.
Data Quality in the Future: Trends and Predictions
As we open the book on the future of data quality, several promising trends emerge that can redefine the data quality landscape.
AI and Machine Learning are set to continue offering significant contributions to data quality. From predicting data quality issues to automating the data cleansing process, these advanced technologies can reduce the time and effort invested in maintaining data quality, while also improving the accuracy and consistency of data.
The cloud is another trend that's impacting the data quality domain. More businesses are moving their data storage and management to the cloud, and this transition requires specialized data quality strategies that match the unique characteristics and challenges of the cloud environment.
Data democratization is a growing trend that affords more employees access to the company’s data. This makes data quality measures even more critical, ensuring that all decision-makers throughout the organization can rely on accurate, consistent, and complete data.
Data privacy regulations are evolving rapidly, and this trend is expected to continue in the future. As new regulations are introduced and existing ones are updated, maintaining data quality will help businesses stay compliant and avoid penalties.
The expansion of IoT (Internet of Things) adds a new dimension to data quality as it brings an avalanche of real-time data. Ensuring the accuracy and timeliness of this data will be a prime focus of future data quality efforts.
These trends provide a window to the future of data quality – a future where data quality is intricately linked with advanced technologies, privacy laws, and business strategies. As the role of data continues to grow in the business landscape, maintaining high data quality will only become more vital. By keeping pace with these trends, businesses can ensure their data quality strategies remain relevant, effective, and future-friendly, paving the way to a successful data-driven future.
If you're interested in exploring how Deasie's data governance platform can help your team improve X, click here to learn more and request a demo.
If you're interested in exploring how Deasie's data governance platform can help your team improve Data Quality, click here to learn more and request a demo.