What Data Quality Means for Your Business Success
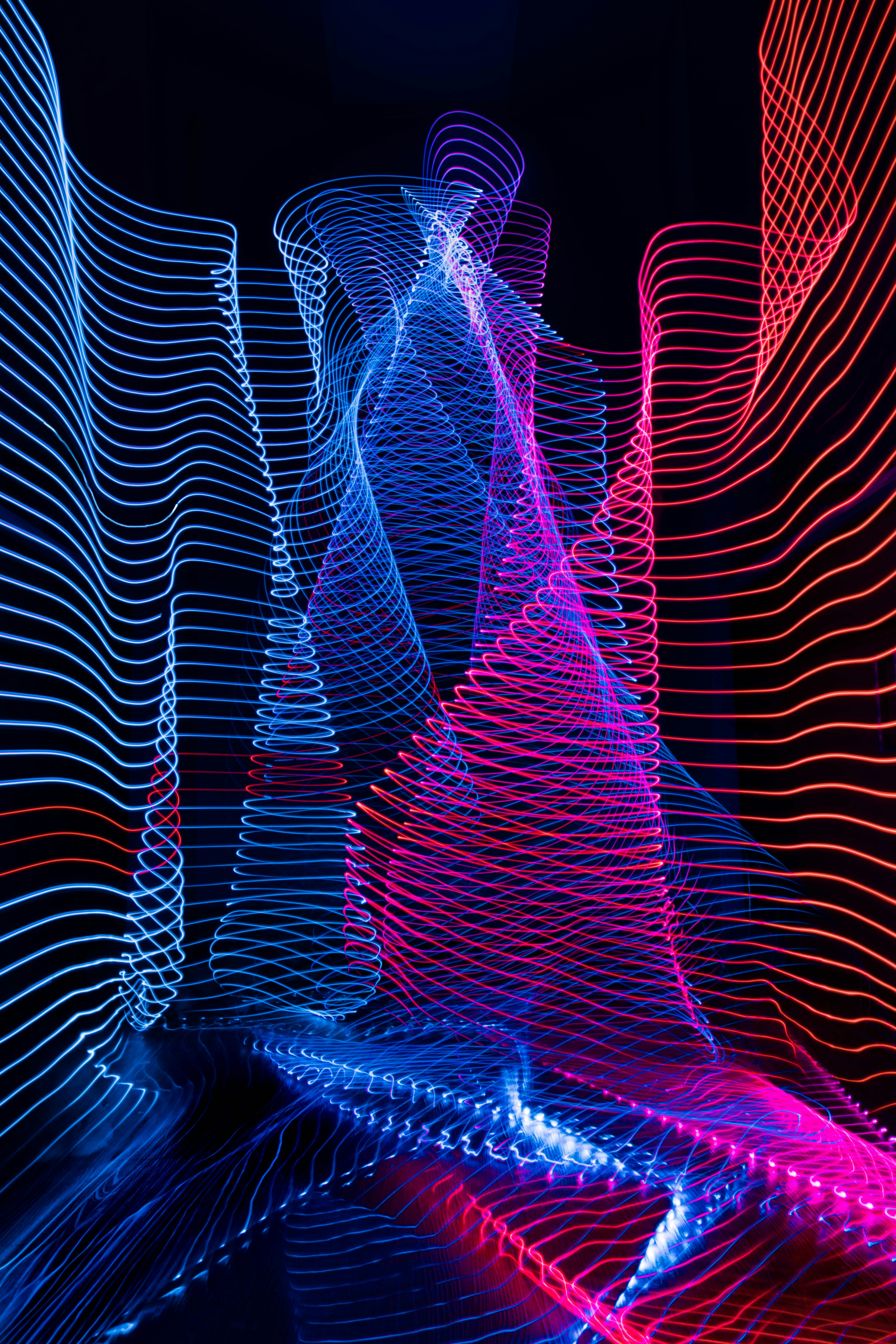
Defining Data Quality
To kickstart our insightful journey into the crux of business success, we need to stride towards a clear understanding of the term 'data quality'. In simplest terms, it's the measure of data's condition matching its intended purpose. But given the complexity and layers of data usage in the modern business landscape, we need to peel back a few more layers of this concept.
If we dive deeper, data quality reflects the overall utility of data by considering various core characteristics. Six fundamental dimensions define high-quality data - accuracy, validity, reliability, timeliness, relevance, and completeness.
Accuracy: An accurate data piece genuinely represents reality. It's free from errors and inaccuracies, thereby allowing businesses to make prudent decisions. Inaccurate data can lead to misguided strategies, ultimately inhibiting business growth.
Validity: It's about shunning square pegs into round holes. Data is valid if it conforms to the predefined rules of your systems. For example, an email field should contain valid email addresses, not phone numbers or names.
Reliability: To call data reliable, it has to stand the test of time. Reliable data is consistent across the system regardless of when and where it is accessed. It brings uniformity to the business operations.
Timeliness: In today's rapidly evolving digital market, outdated information is as detrimental as having no data at all. Timely data enables enterprises to respond effectively to changes and maintain a competitive edge.
Relevance: Every data piece recorded should serve a purpose that aligns with your business objectives. Irrelevant data, no matter how accurate or timely, only contributes to information overload without offering tangible value.
Completeness: No jigsaw puzzle can be completed if pieces are missing. Similarly, incomplete data is often misleading and hinders comprehensive business analysis. It's pertinent to have the entire dataset to gain a holistic, accurate view.
Each of these dimensions brings light to a different aspect of data quality, contributing to the holistic perspective needed for a successful data-driven strategy.
The Role of Data Quality in Business
As we move deeper into the era of digital transformation, enterprises are increasingly harnessing the power of data to drive innovation, growth, and success. Let's delve into how data quality factors into such pursuits.
Optimizing Operational Efficiency: In the modern business world, well-managed data acts as the oil that keeps the corporate engine running smoothly. High-quality data paves the way for seamless business processes, eliminates redundancies, and fosters efficiency. It aids in cost reduction by minimizing errors that create bottlenecks, thus improving overall operational efficiency.
Empowering Decision Making: High-quality data equips businesses with the insights needed for sound decision making. It provides an accurate representation of market trends, customer preferences, and competitive landscapes, enabling businesses to make decisions backed by solid, reliable information.
Enhancing Customer Experience: Today, delighting customers has moved beyond just offering exemplary products or services; it involves delivering experiences. Accurate and timely customer data allows businesses to understand individual needs, tailor experiences, and build lasting relationships, thus significantly enhancing customer satisfaction and loyalty.
Ensuring Regulatory Compliance: Industries like financial services, healthcare, and government operate under strict regulatory constraints regarding data management. High-quality data, combined with effective management practices, ensures organizations remain compliant, mitigating the risk of legal complications or penalties.
Data quality is like a compass for businesses navigating the sea of digital transformation. It helps steer the ship towards the shores of success, with its role magnifying at each step of the journey. Well-curated and managed data is not just a resource; it's a strategic asset driving business growth in today's data-driven world.
How Poor Data Quality Impacts Your Business
In the panorama of data-driven enterprise operations, the ebbing specter of poor quality data is as perilous as navigating through a storm without navigation instruments. The implications it can have on an organization are multi-fold, enveloping various facets of enterprise functionality.
The High Cost of Poor Quality Data: Data of poor quality is like an invisible anchor, pulling down your business pursuits. It's an often underestimated cost center, causing businesses to pay dearly in wasted resources, missed opportunities, and poor decision-making. A subtle typo in an address field can lead to shipment errors, causing supply-chain disruptions and disappointed customers.
Negative Effects on Customer Relationships: Just like rust on metal, poor quality data silently erodes the foundation of customer relationships. Erroneous customer data can lead to miscommunication, poor customer service, and a breach of trust. When businesses lose sight of customers' actual needs due to inaccurate data, they risk damaging relationships, losing loyalty, and, ultimately, profitability.
Issues with Scalability and Automation: Poor data quality is a roadblock in the path toward business automation and scaling. Automating processes with underlying data issues only serves to magnify those problems, translating them into amplified business errors. On the other hand, data quality issues can delay scaling initiatives as businesses might need to clean up their data before they can proceed with expansion plans.
Risk of Non-compliance: We outlined earlier how good data quality can help in maintaining regulatory compliance. Still, it's equally important to understand the potential repercussions of poor data hygiene. In sectors with stringent compliance regulations, inaccurate or incomplete data can lead to infractions, heavy penalties, and can tarnish the reputation of an organization.
Data Quality and Advanced Analytics
"Eureka!" – that jubilant feeling when a critical business insight is uncovered through analysis. However, one must remember that the insights derived are only as excellent as the underlying data quality. In the realm of advanced analytics, poor data quality is like a fog that obscures the paths to valuable insights.
The Role of Data Quality in Machine Learning & AI: Machine Learning and AI rely heavily on training data to learn, adapt, and generate outputs. If the underlying data used for algorithm training is inaccurate or incomplete, the models may develop erroneous understanding, leading to misguided outputs. Thus, data quality is an absolute necessity to build robust, reliable algorithms in machine learning and AI.
Effects on Predictive Models & Algorithms: Predictive models that are fed low quality data are bound to make inaccurate forecasts. The precision and reliability of such models and algorithms are, to a great extent, determined by the quality of the data driving them. Clean, high-quality data can help businesses take full advantage of advanced analytics tools, predictive modeling, and automated decision-making.
Influence on Data Usability & Accessibility: Data with high volume but low veracity acts more like a labyrinth than a goldmine. High-quality data promotes usability and accessibility, enabling data scientists and analysts to extract valuable insights rather than investing time in data cleaning and preprocessing.
Data quality forms the foundation upon which advanced analytics stands. The way data is captured, stored, processed, and maintained can make a significant difference in an organization's analytical capabilities and, by extension, its competitive advantage in the data-driven business landscape.
Steps to Improve Data Quality
Cognizance of the need for high-quality data is only the beginning; taking proactive steps towards its improvement is the next vital phase. Ensuring excellent data quality need not be an overwhelming endeavor but rather an ongoing commitment to a set of strategic initiatives.
Implementing a Data Governance Framework: A well-structured data governance framework aligns everyone in the organization towards common data handling protocols, thus improving overall data quality. It helps define data, set standards, assign responsibilities, and manage changes to ensure accuracy, consistency, and security at all times.
Adopting Data Quality Management Tools: Venturing into data quality improvements without the right tools is akin to sailing without a compass. Data quality management tools aid in detecting and correcting errors, automating data cleansing, validating and monitoring data, and offering detailed insights to understand where improvements are needed.
Regular Data Auditing & Cleansing: Regular audits enable businesses to keep a close eye on the pulse of their data's health. It aids in timely identification and rectification of inconsistencies and inaccuracies. Integrating data cleansing in routines helps maintain clean, usable data by removing, correcting, or replacing corrupt or inaccurate parts of data.
Incorporating a Culture of Data Quality: Above all, fostering a data-conscious culture helps uplift data quality. A culture that acknowledges the importance of accurate, complete, and timely data encourages everyone to do their part in improving and maintaining high data quality.
Case Studies of Successful Data Quality Management
In our orbit around data quality and its implications, it's enlightening to bring into spotlight some real-world examples of enterprises that have harnessed the power of high-quality data.
Successful Data Quality Management in Healthcare: The healthcare industry stands as a testament to the wonders of good data quality management. Implementing stringent data governance policies, regular data audits, and sophisticated data management tools enabled these organizations to record, track, and analyze patient data more accurately. This accuracy played a pivotal role in enhancing patient care, decreasing administrative errors, improving service efficiency, and ultimately saving lives.
Data Quality Triumphs in the Financial Services Sector: Financial institutions face continuous pressure to deliver accurate and timely data—a single error could mean a loss of millions or breach of compliance regulations. Embracing proactive data quality measures coupled with machine learning techniques, financial service firms have been able to drastically improve their data accuracy and reliability. This improvement has streamlined their decision-making process, enhanced risk management, increased operational efficiency, and bolstered customer relationships.
Government Sector and Embracing Data Quality: The public sector, too, hasn't shied away from reaping the benefits of good data quality. Government departments dealing with population data, social security, and law enforcement have implemented systematic data quality measures. The result has been an increase in citizen engagement, improved service delivery, accelerated decision-making, and enhanced transparency and trust.
These real cases underline the power held by data quality. Achievements across different sectors only mirror the potential success enterprises from all walks of life can expect when they enhance their focus on data quality.
If you're interested in exploring how Deasie's data governance platform can help your team improve Data Governance, click here to learn more and request a demo.