Unstructured Data Solutions: Addressing the Challenges of Complex Datasets
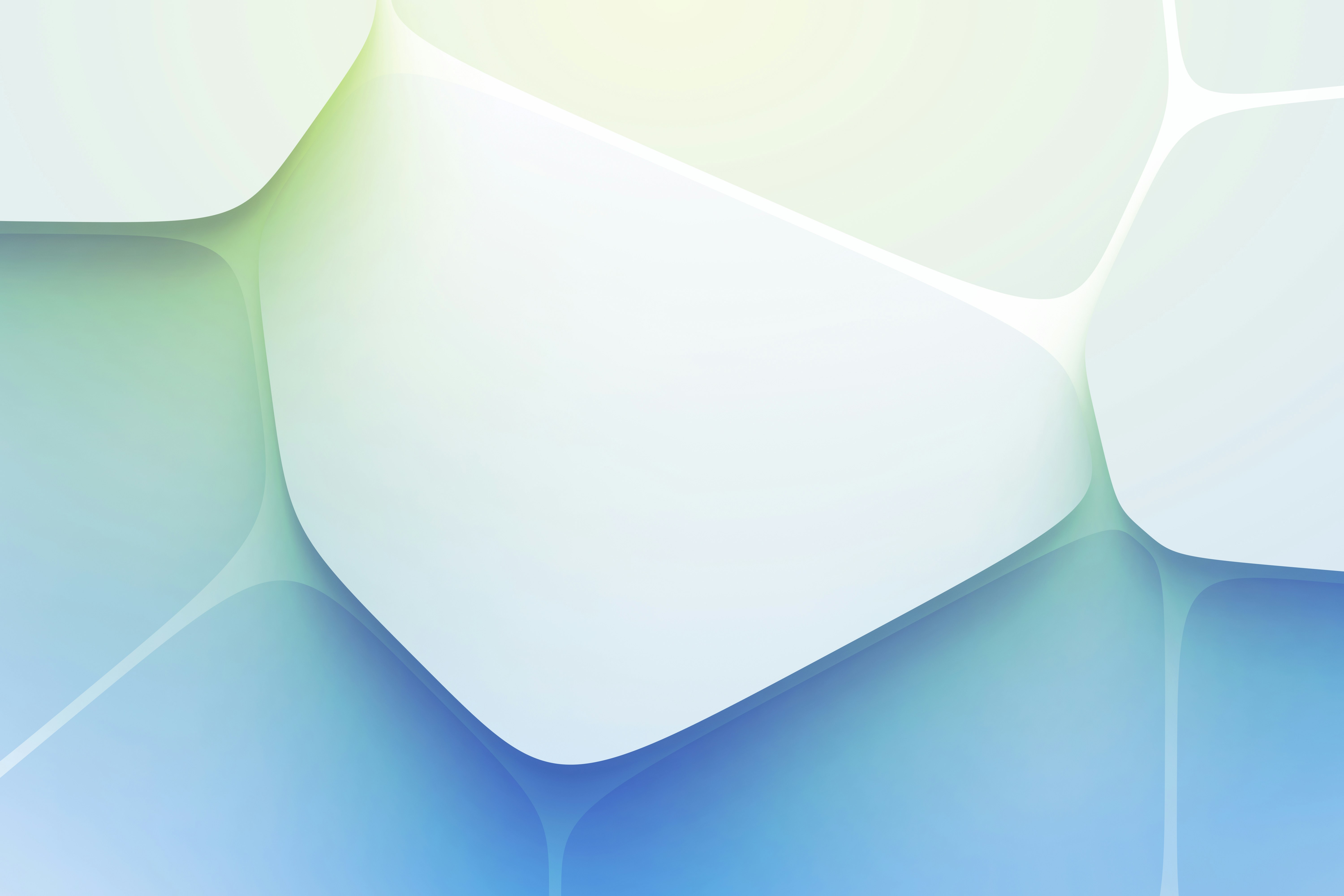
Understanding Unstructured Data
In the realm of data analysis, unstructured data can be simultaneously boon and bane. It represents the vast majority of available data, spanning social media posts, business documents, emails, media files, and more. Defined by its non-conforming nature to pre-determined formats or models, unstructured data has the potential to offer a treasure trove of insights that aid decision-making in organizations. Companies that harness and manage this data source efficiently stand to gain substantial competitive advantages.
The importance of managing unstructured data is underpinned by its potential for critical business insights. These could range from customer sentiment analysis and market trends to internal reporting patterns and decision-making behaviors. Effectively processed and analyzed unstructured data can offer leading-edge insights, allowing large enterprises to identify early market shifts and maintain an advantage over competitors.
However, the very richness of unstructured data contributes to the challenges associated with it. Its scale, diversity, and absence of a predefined model make analysis complex and resource-consuming. Storing, managing, and synthesizing disparate data types becomes a puzzle, while large volumes can overwhelm traditional data management tools, slowing down processes and risking data loss or inconsistency.
The Role of Machine Learning & AI
Meet machine learning (ML) and artificial intelligence (AI), the cutting-edge technological allies that led a revolution in unstructured data management. By leveraging learning algorithms and analytical models, ML and AI can parse, understand, and structure vast volumes of unstructured data quickly and accurately. This advanced data management serves to unlock the hidden value in unstructured data, facilitating deeper analysis and more potent business insights.
ML and AI outmatch traditional techniques when dealing with unstructured data. Powered by diverse learning algorithms, these technologies can perform tasks like keyword spotting, sentiment analysis, trend tracking, and entity recognition, among others. In healthcare, digital medical records can be parsed to extract vital data points that can aid in diagnosis and personalized care. In finance, customer communication can be analyzed to refine marketing strategies or detect potential fraudulent activities.
It is worth noting that ML and AI technologies go beyond data extraction and analysis. They also offer predictive modeling capabilities, using analyzed data to forecast future trends or behaviors. For instance, social media posts can be scoured to predict public response to a new product launch. As such, ML and AI serve not just to manage unstructured data but also to furnish future-facing enterprises with a predictive edge.
GenAI's Application for Unstructured Data Solutions
In the midst of an explosive data landscape, Generative AI (GenAI) has emerged as a powerfully effective mechanism to deal with scenario complexity and high-volume data conundrums. With an inherent ability to derive patterns from big data and generate new data points that mimic the provided input, GenAI provides a uniquely adaptive solution for managing unstructured data. This capability is complemented by GenAI's infrastructural elasticity, allowing it to scale up or down in response to varying data loads, a crucial feature for large enterprises handling a range of data volumes.
Large Language Models (LLMs), as a crucial component of GenAI, play a direct role in deciphering and managing unstructured text data. They leverage their extensive training on various bi-lingual tokens to generate contextually aware responses, which greatly aids in data understanding and inference. This particular feature is crucial in scenarios where the LLMs are further fine-tuned with specific industry data, enhancing the model's ability to provide useful insights.
When deployed effectively, GenAI can offer real-world positive outcomes to enterprises. With its ability to interpret vast amounts of data and learn from it, companies can peek into data-driven insights that were previously unreachable. Be it predicting market trends to stay ahead of the competition, identifying anomalies in financial transactions to tighten security, or deriving diagnostic insights from patient records to enhance healthcare services, GenAI becomes a transformative tool in an organization's arsenal.
Deep-dive into GenAI Enhanced Techniques
Retrieval Augmented Generation (RAG) acts as a particularly lucrative function when managing unstructured data with GenAI. At its core, RAG retrieves and assimilates external information into the LLM's prompts to allow the model to output responses that reflect unique contexts - a process that opens a wide-ranging set of applications for the GenAI models.
When dealing with unstructured data, RAG becomes instrumental. Given that unstructured data is expansive and diverse, situating data in a specified context becomes critical for Z-relevance and meaning. RAG, with its ability to retrieve and introduce external information, addresses this issue significantly.
RAG’s application extends to a host of real-world use cases. For instance, customer service bots powered by LLMs enhanced with RAG can pull in real-time, external data to provide the most informed, contextually accurate support. In literature analysis, RAG can introduce related reference materials or prior analyses to give more depth to the model's generated review. Lastly, in fraud detection, the augmentation technique can fold-in recent fraud patterns to better equip the model to spot potential risks.
Appropriateness of these Solutions for Regulated Industries
GenAI's prowess in sifting through, analyzing, and drawing insights from unstructured data makes it particularly suitable for heavily regulated industries, which routinely grapple with vast data sets.
In financial services, for instance, banks and investment firms deal with a profusion of unstructured data daily, ranging from transaction data, customer communication, market news, and more. Here, GenAI, coupled with RAG, can be invaluable for tasks such as sentiment analysis from customer communication for enhanced service delivery, or the identification of fraudulent behavior by detecting anomalies within transaction data.
Healthcare is another domain where AI and ML techniques come to the rescue. Given the abundance of patient data in the form of narratives, medical records, images, and more, applying GenAI to this wealth of unstructured data can lead to finer health insights, better diagnosis procedures, and personalized medical care. Furthermore, the integration of large language models can enable complex risk assessment by analyzing patient history, potentially saving lives by predicting health risks.
Government agencies, too, are awash with unstructured data in the form of policy documents, reports, citizen feedback, among others. GenAI's capability to extract context, meaning, and insights from such data can streamline public services, enhance policy-making, and amplify citizen engagement through improved communication.
Overcoming Challenges with GenAI-powered Unstructured Data Solutions
Despite the enormous potential of GenAI and RAG, their use comes with inherent challenges that must be addressed responsibly. Some limitations involve technical aspects such as the requirement of large datasets for effective GenAI training and the difficulty in controlling the outputs of LLMs resulting in potential biases in generated responses.
Managing these requires a strategic combination of robust technological handling and guiding regulations. In cases where data availability can be a hindering factor for GenAI implementation, techniques such as data augmentation or synthetic data generation might help create sufficient training material. To curb biases, stringent post hoc filtering processes and continuous alignment with human values can ensure outputs are accurate and impartial.
Risk mitigation in GenAI deployment should not be overlooked. Given these tools interact with large amounts of data, often sensitive, their use must be governed by robust privacy and security regulations. This is particularly critical for industries handling sensitive data, such as healthcare or finance. Anomalies and errors should be continually monitored and addressed promptly to maintain the integrity and safety of the data involved.
In conclusion, acknowledging and addressing these challenges does not diminish the importance of GenAI and RAG in unstructured data management, but reinforces our responsibility in deploying such powerful tools with due diligence and caution. The benefits of implementing such solutions, from business optimization to service improvement, easily outweigh these addressable challenges. With the right strategies and careful monitoring, GenAI can continue to revolutionize unstructured data handling for the better.
The Future of Unstructured Data Solutions
Unstructured data management stands at the dawn of an exhilarating era - one primed to be steered by the powerful strides in GenAI and LLM capabilities. The advancements predict a dominant trend of increasingly effective, fast, and nuanced tools and techniques that make unstructured data handling less of an uphill task and more of an insightful undertaking.
There is a growing recognition of the role that GenAI and LLMs will play in influencing the transformation of data-dependent sectors. These technologies will continue to shape the data landscape, enabling large enterprises and regulated industries to find value amidst the data deluge. The future will usher in even more innovative applications of these techniques, making unstructured data a less daunting possibility and more of a valuable business resource.
Moreover, businesses need to remain agile and willing to adapt to these evolving technologies. Encouraging cross-departmental collaboration, investing in employee training, and revisiting data strategy will be essential steps in preparing businesses for this new GenAI-driven information management era. Equipped with the right tools, businesses can navigate through unstructured data with precision and be ready to harness the insights that emerge. While challenges will exist, an unwavering pursuit of technical progress, regulatory diligence, and ethical vigilance will guide us to a future where unstructured data ceases to be an obstacle but a competitive advantage.
If you're interested in exploring how Deasie's data governance platform can help your team improve Data Governance, click here to learn more and request a demo.