Unstructured Data Problems: Overcoming Common Challenges
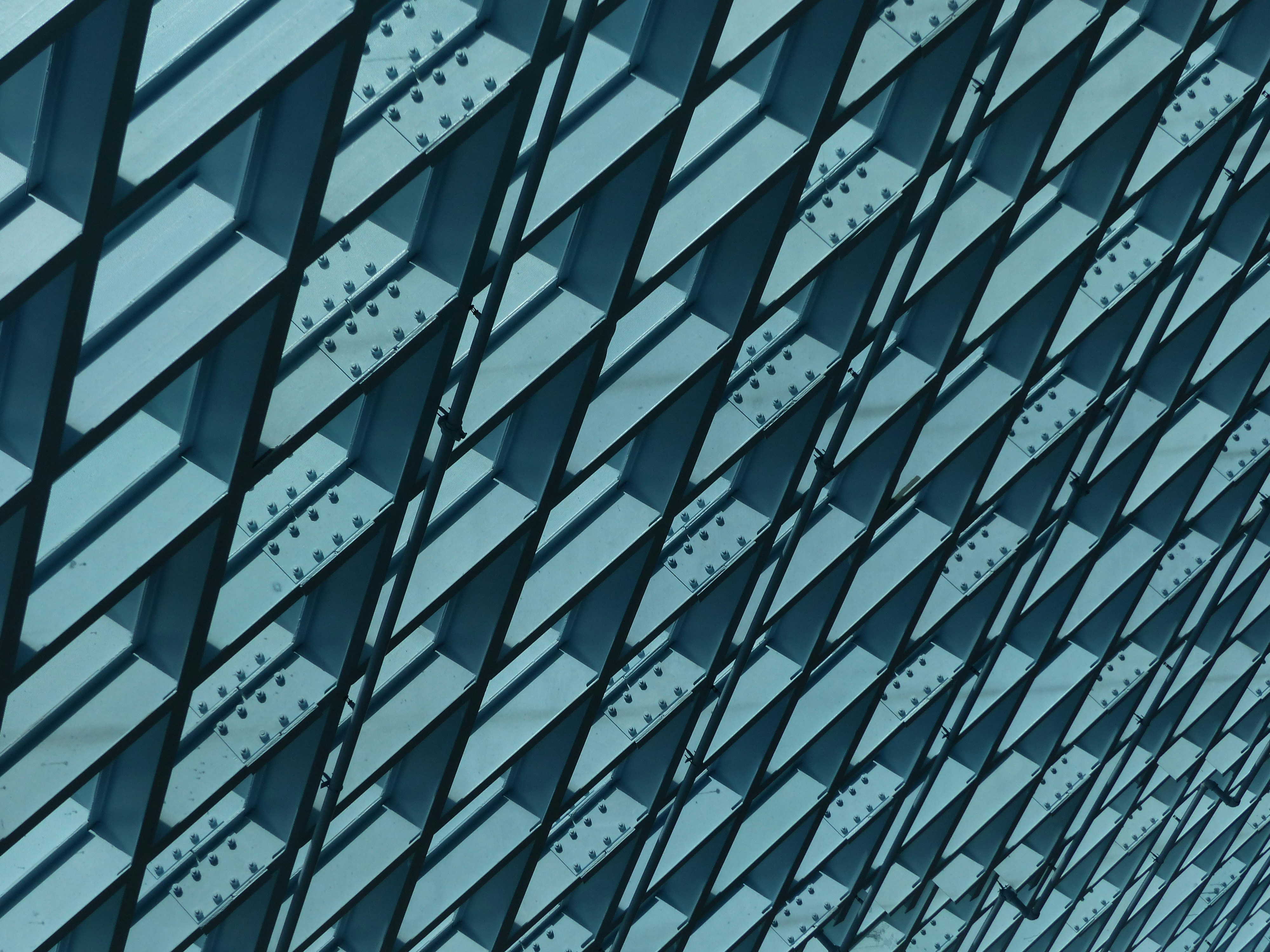
Defining Unstructured Data
Unpredictable and unorganized, unstructured data is the unidentified hero of valuable business insights. Unstructured data isn't typically organized in a pre-defined manner or possess an identifiable structure. Examples span an extensive array; from emails and word documents to images, video and social media exchanges. Essentially, it’s the information that doesn't fit neatly into databases or spreadsheets.
Amid the growing surge of digitalization, unstructured data has become an unprecedentedly significant facet of business operations. Whether emerging from business processes, digital engagements or external interactions, they contain gems of information, capable of transforming strategic decisions.
Despite it's disarrayed exterior, unstructured data forms a substantial percentage of enterprise data, estimated at a staggering 80-90%. Let this sink in - the majority of a company's potentially invaluable data pool is unstructured, often untouched due to its elusive form. Harnessing this untapped potential can invariably catapult businesses ahead of their competition, making it an essential aspect of strategic planning.
Challenges with Unstructured Data
Unstructured data's diverse origins and forms present several challenges that need addressing. Let’s delve into these issues and understand their strikes on the optimal utilization of unstructured data.
Inefficient Data Management Navigating through the sheer volume of unstructured data can resemble finding a needle in a haystack – tedious and often overwhelming. The lack of standardized formatting makes data indexing, storing, retrieving, and managing a challenging endeavor.
Data Security Concerns Guarding unstructured data with stringent security protocols is not as straightforward as with structured data. Its numerous forms and sources, coupled with its storage across various platforms, dictate a need for diverse and dynamic security applications, making the task considerably complex.
Difficulty in Data Integration Integrating unstructured data with the structured data resources in an organization is complicated. This is due to the polymorphic nature of unstructured data that doesn’t conform to a specific form or function.
High Costs of Data Storage The exponential growth of unstructured data commands considerable storage. Given that traditional methods of managing and storing data are ill-suited to handle the explosion of unstructured data, businesses often face the hurdle of high storage costs.
Each of these challenges can impede an organization’s ability to access, interpret, and gain valuable insights from unstructured data. It's a massive roadblock, considering unstructured data's potential to harbor insights that could drive strategic decision-making and operational efficiency.
The Significance of Unstructured Data in Machine Learning & AI
The kinetic world of Machine Learning (ML) and Artificial Intelligence (AI) has found a goldmine in unstructured data. Driven by the data-dependent foundation of ML and AI, unstructured data lie at the crossroads of insightful business intelligence and advanced technology.
Unstructured data feeds into the predictive models of ML, shaping its ability to make informed future predictions. Passenger drones, for instance, use unstructured data like weather conditions and route mapping, to train flight navigation algorithms.
The enigmatic world of AI finds deciphered codes in unstructured data too. The functional abilities of AI to understand, interpret, and respond are trained predominantly by unstructured data. Take, for example, customer service chatbots. Their ability to contextually answer customer queries has been trained using unstructured data in the form of pre-existing customer interactions.
Thus, unstructured data furnish a rich, varied training set for ML algorithms while enhancing the cognitive abilities of AI applications. A mutual exchange where unstructured data drives AI and ML, creating lean applications that can further process unstructured data.
Leveraging AI and ML to Solve Unstructured Data Problems
AI and ML are not just the beneficiaries of unstructured data—they come full circle to provide comprehensive solutions for handling it.
AI stands at the forefront to extract understanding from the vast chaos of unstructured information. Techniques like natural language processing (NLP) allow AI to comprehend human language in the form of text data. Machine learning algorithms trained to identify patterns are widely used to mine unstructured data for meaningful insights.
For instance, sentiment analysis, a popular application of AI, can scan through social media posts and online reviews, providing insights into consumer behavior and market trends. Similarly, in healthcare, AI can interpret unstructured data like radiology images to support medical diagnoses.
Machine Learning underpins the ability to classify, organize, and understand unstructured data. With training data as an initial input, ML algorithms can identify patterns and predict outcomes. They can be deployed to categorize data, detect anomalies, or predict trends. From discerning a potential malware attack from network logs to proactive customer support through email analysis, ML casts a wide net in addressing unstructured data problems.
These concepts are just a glimpse into the vast realm where Machine Learning and Artificial Intelligence operate, continually evolving to manage unstructured data better. Their potential is far from being fully exploited, creating an exciting space of ongoing progress and remarkable problem-solving capabilities.
Key Tools and Techniques to Handle Unstructured Data
Navigating unstructured data's vast expanse can seem daunting. Fortunately, several advanced tools and techniques can aid businesses in managing and interpreting this form of data.
Text Analytics and Natural Language Processing (NLP) Unstructured text data is one of the most prevalent types; everything from emails and social media posts to customer reviews falls under its umbrella. NLP and text analytics are pivotal for decoding this information. They provide structure to text by identifying entities, extracting attributes, and understanding the sentiment, which aids in data categorization and extraction of insights.
Image and Video Analytics AI technologies, such as computer vision, are indispensable for interpreting image and video data. They can identify trends, categorize images and even link visual content with relevant metadata. This could be useful for any business, from retail utilizing visual search algorithms to healthcare utilizing diagnostic assistance in radiology.
Data Mining and Pattern Recognition Through algorithms, pattern recognition and data mining techniques can decipher patterns and correlations within sprawling datasets. They help businesses identify trends, make predictions, and guide strategic decision-making.
Real-world Use Cases of AI & ML in Managing Unstructured Data
Unstructured data, when leveraged correctly, can deliver tangible results. Here are a couple of instances where industries have successfully employed AI and ML to manage their unstructured data.
Healthcare: Medical Image Interpretation Radiology images are a prime example of unstructured data in healthcare. AI can analyze these images, detect patterns, and provide diagnostic assistance. For example, machine-learning algorithms can identify early signs of diseases like cancer, improving prognosis and treatment outcomes.
Financial Services: Sentiment Analysis for Market Trends In the volatile world of finance, understanding market sentiments can be crucial. Financial institutions use sentiment analysis, a tool powered by AI and ML, to derive insights from unstructured data like news articles, social media posts, and financial reports. These insights can forecast market trends and guide investment strategies.
Government: Enhancing Public Safety In the public sector, predictive policing models use unstructured data like CCTV footage, crime reports, and social media posts to anticipate potential crime hotspots. This aids in resource allocation and proactive law enforcement for increased public safety.
These examples illustrate how the fusion of AI, ML, and unstructured data is shaping industries, enabling innovative solutions and business practices. With technology progressing every day, the potential implications are vast and unexplored.
If you're interested in exploring how Deasie's data governance platform can help your team improve Data Governance, click here to learn more and request a demo.