Unstructured Data Is Best Defined As: Understanding Complex Information
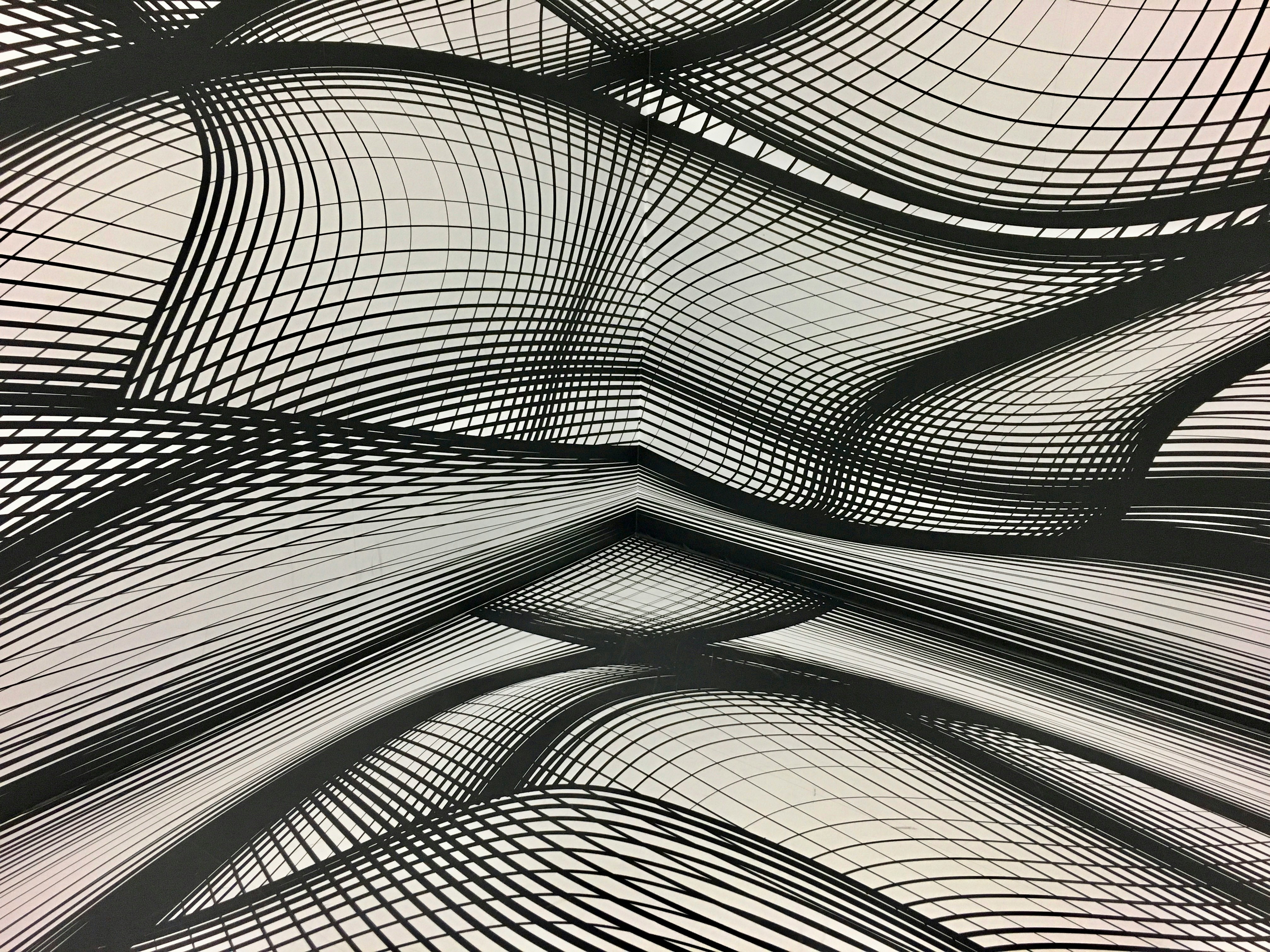
Defining Unstructured Data
When we talk about data, we frequently refer to structured information, which fits neatly into organized fields in databases or spreadsheets. Examples include names, phone numbers, or IDs—all easily searchable, identifiable pieces of data that machines find uncomplicated to handle.
But when it comes to unstructured data, the scenario changes significantly. Unstructured data is heterogeneous information that doesn't adhere to a pre-defined model or isn't easily searchable. It could range from text files, social media posts, emails and business documents, to even more complex forms like podcasts, videos, and satellite imagery. The key characteristic of unstructured data is its lack of structure—it doesn't fit into conventional data models and is resistant to common data processing techniques.
Understanding unstructured data is crucial because it holds a vast amount of invaluable insights—often more insightful than structured data. Uncovering patterns, connections, and trends in this sea of information can offer enterprises a competitive edge and a comprehensive viewpoint on various business areas. The challenge, however, resides in the extraction, interpretation, and utilization of this scattered and complex information, which traditional analytic models and algorithms find hard to handle.
Diverse Types of Unstructured Data
Let's dive a bit deeper to understand the different forms this unstructured data takes:
- Textual Unstructured Data: A dominant form of unstructured data is text-based. This comprises of email communications, PDF files, word documents, social media posts, URLs, and more. Textual information is pervasive and contains valuable insights, but its inconsistent formats, diverse topics, slang, typos, and different languages make it a complex field for analysis.
- Non-Textual Unstructured Data: Beyond text, unstructured data expands to visual and audible formats. Audio data includes phone calls, voice recordings, podcasts, radio broadcasts, etc. Video data encapsulates movies, CCTV footage, online streams, and video files. Furthermore, image data refers to photos, pictures, logos, diagrams, charts, maps, X-rays, and similar content. Additionally, there's also sensor data, which includes information from IoT devices, telemetry, biometrics, etc.
The latter forms of unstructured data—non-textual—pose even greater challenges. For instance, to analyze a company's pictorial logo, an algorithm would need to understand colors, shapes, symbols, and even implied meaning—tasks far more complicated than handling structured numerical data. Equally complex is audio data that requires converting sounds, accents, and spoken language nuances into analysable data.
Navigating this landscape is complicated but crucial, as unstructured data, in its many forms, holds untapped potential for many businesses across industries. The key is understanding and leveraging the right tools and strategies to tackle this complexity.
Role of Machine Learning and AI in Unstructured Data Analysis
Unlocking the insights hidden within unstructured data zones is where the power of machine learning (ML) and artificial intelligence (AI) comes into play. ML and AI are designed to handle complexity, understand context, learn from experience, and tackle vast volumes - precisely the challenges that unstructured data brings to the table.
Natural Language Processing (NLP), an exciting field within AI, works towards making sense of textual unstructured data. Through techniques like sentiment analysis, topic modeling, entity extraction, and more, NLP can pull insights from social media posts, customer reviews, research documents, and other textual data. For instance, sentiment analysis can help decode customer emotions from product reviews, while topic modeling can quickly summarize lengthy documents.
For non-textual unstructured data, we have specialized fields within AI such as Computer Vision for images and video, and audio analysis for sounds. Computer Vision decodes images and videos to identify objects, classify visual content, or detect anomalies. A retail outlet might, for instance, use computer vision to analyze CCTV footage for customer behavior or shoplifting attempts.
Deep Learning, a subset of ML, has also proven remarkably useful in processing unstructured data. From convolutional neural networks (CNNs) for image recognition to recurrent neural networks (RNNs) for sequence analysis, these advanced techniques make unstructured data analysis more accurate and efficient.
Handling Large Volumes of Unstructured Data
The volume of unstructured data that businesses generate and collect daily is massive. Photos are uploaded on social media, emails are sent, CCTV cameras capture footage - every second, amassing colossal amounts of unstructured data. Handling this data influx is a significant challenge but also an opportunity.
This area is where modern data processing methodologies come into the picture. Distributing data processing across several servers or nodes, techniques like MapReduce can handle and analyze huge data volumes efficiently.
Then enters the era of cloud solutions, reshaping how businesses store and manage unstructured data. Cloud storage and processing technologies offer scalable, flexible, and cost-effective solutions for handling such data. Storing unstructured data in the cloud is not only more affordable but also paves the way for advanced cloud-based analytics and AI tools to extract maximum insights from this data.
Data lakes are another solution gaining traction to store large volumes of raw, unstructured data. Unlike traditional databases that require a defined structure, data lakes allow storing data in its native format, ready for future analysis.
The handling of large volumes of unstructured data is a complex process, but with the right techniques and tools, it becomes a manageable task. This approach not only preserves the data's integrity but efficiently turns it into useful, actionable insights.
Real-World Applications and Use Cases
Unstructured data has penetrated various industries, and understanding its value can be best observed through real-world applications and use cases.
In financial services, firms deal with vast amounts of unstructured data daily - financial reports, market news, customer emails, transaction records, and more. By employing AI and ML, these institutions can extract valuable customer insights, predict market trends, detect fraudulent activities or optimize their operational effectiveness.
Healthcare is another sector where unstructured data shines via medical images, health records, doctor's notes, research data, and patient feedback. Advanced AI technologies can interpret these data points, improve diagnostic accuracy, predict patients' health risks, personalize treatments, and ultimately drive more robust and efficient patient care.
Government organizations, despite dealing in largely structured information, also produce and encounter a large amount of unstructured data - public feedback, policy documents, satellite images, census data, etc. Analysis of this data provides valuable insights for strategic planning, policy designing, resource allocation, and even enhancing public safety and security.
Across these sectors, and more, unstructured data, when correctly harnessed, illuminates paths towards better decision-making, efficiency, customer satisfaction, and substantial competitive advantages.
The Future of Unstructured Data Analysis
Projecting into the future, the role of unstructured data in enterprises is set to amplify multifold. Predictive analytics and Big Data techniques will enable businesses to harness unstructured data to anticipate future trends, consumer behavior, and market dynamics. As our world becomes increasingly digitized, the data we produce will also grow in volume and complexity. Unstructured data is poised to play a pivotal role in shaping these futuristic insights.
Further, in today's AI-powered era, the tools and solutions for analyzing unstructured data are constantly evolving. From automated data extraction to intelligent analytics, the revolution in AI and ML capabilities will continue to drive unprecedented insights from unstructured data.
The path forward lies in the integration of these technologies into the data strategy of organizations—realizing that unstructured data is not just noise but a treasure trove of untapped potential. As businesses navigate the unstructured data terrain, the distinguishing factor will be the coupling of the right technologies, strategies, and a dose of innovative thinking.
If you're interested in exploring how Deasie's data governance platform can help your team improve Data Governance, click here to learn more and request a demo.