Unstructured Data in Healthcare: Innovations and Challenges
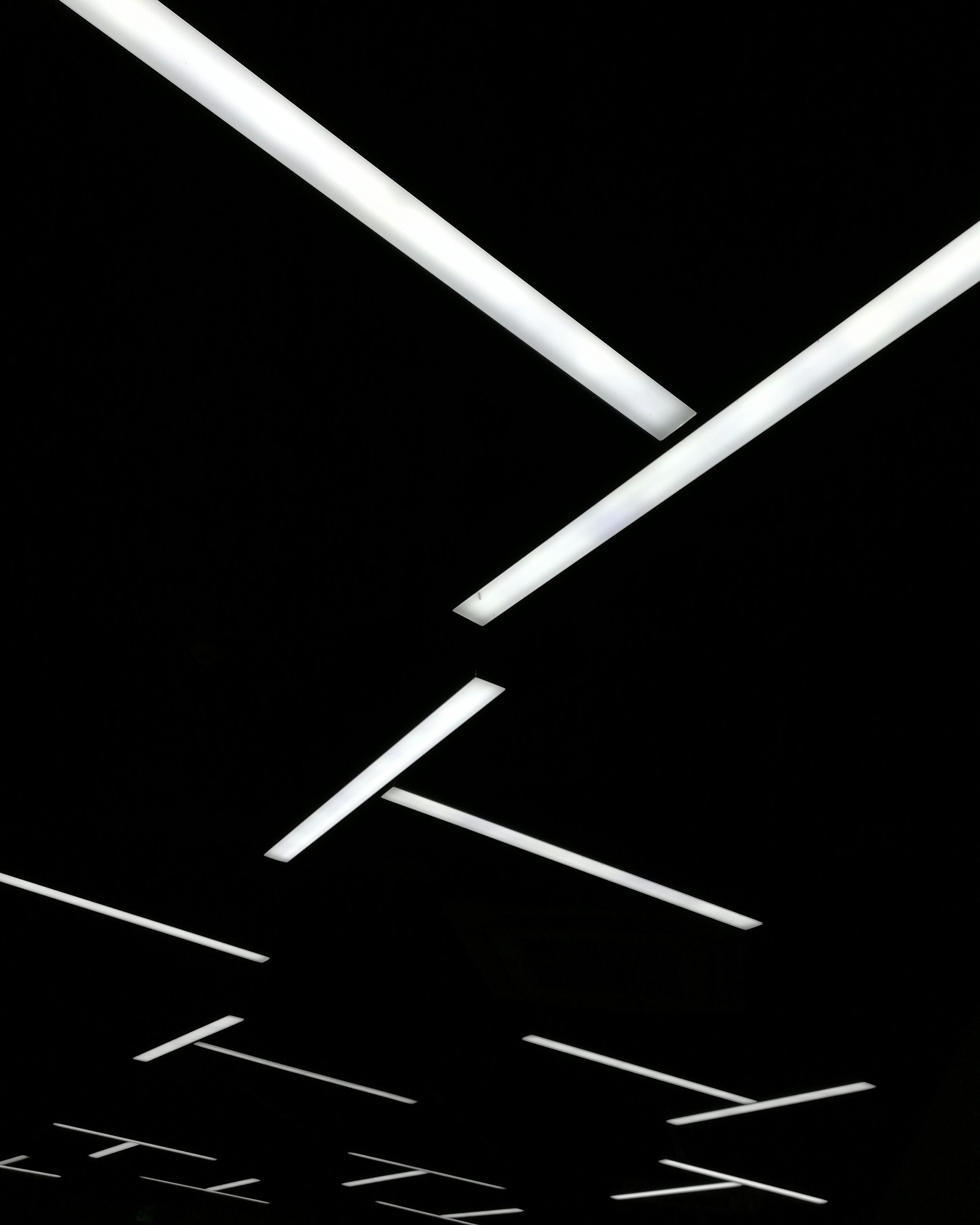
Understanding Unstructured Data in Healthcare
In the world of healthcare, the goldmine of data is substantial and continuously growing, with one particular kind standing out: unstructured data. This term refers to information not organized in a pre-defined manner or does not have a pre-defined data model. Unlike structured data that neatly fits into tables or excel spreadsheets, unstructured data is more free-form. It encompasses medical notes written by healthcare professionals, emails between doctors and patients, imaging data, laboratory results narratives, audio dictation recordings, and health history narratives. Understanding the full breadth and depth of unstructured data is the first step in unlocking its potential.
The impact of unstructured data in the healthcare arena can't be understated. It plays a crucial role in every patient's journey from diagnosis through treatment and ongoing management. Unstructured data generated during these interactions often contain valuable insights that can enhance decision-making processes and patient outcomes. By bridging the gap between complex medical histories and care delivery, unstructured data serves as a rich source of information for healthcare providers, researchers, and caretakers.
Challenge of Managing Unstructured Data
Despite the immense potential, unstructured data in healthcare often brings considerable challenges to the table. Firstly, the sheer volume and complexity of unstructured data can be staggering. With the digitization of healthcare records, there's an explosion of data from various sources every day. From digital imaging to electronic health records (EHRs) and wearable device outputs, all these add to the extensive pool of data, giving rise to issues in storage, management, and accessibility.
Secondly, maintaining the quality and consistency of unstructured data is a labor-intensive task. Given its diverse sources and the absence of a standardized format, unstructured data can vary significantly in completeness, accuracy, and relevance. For healthcare providers aiming for precise and informed decisions, the inconsistent quality can pose hurdles in generating insightful analysis.
Lastly, the analysis and integration of unstructured data can be taxing. Standard data analysis tools are usually not equipped to handle the nuances of unstructured data. Thus, translating these massive, complex datasets into usable clinical insights is hugely challenging. Moreover, integrating unstructured data with structured data to provide a comprehensive view of a patient’s health can pose unique technical difficulties.
Despite these challenges, managing unstructured data is not an insurmountable task, especially with innovations brought by AI and machine learning.
Innovations in Managing Unstructured Data in Healthcare
Harnessing the power of artificial intelligence (AI) and machine learning (ML) opens doors to convert unstructured data's obstacles into opportunities. These technologies have shown a substantial capacity to manage, analyse and draw meaningful patterns from these extensive, multi-format datasets.
Natural Language Processing (NLP), a subfield of AI, is particularly significant in dealing with textual unstructured data like patient notes, medical reports, and emails. NLP algorithms can break down, understand, and derive meaning from human language, facilitating the conversion of textual data into structured, analysable formats.
Deep learning, another subset of machine learning, shines in handling imaging data, an integral part of unstructured data in healthcare. Deep learning models excel in interpreting images, helping identify disease patterns in scans and improving the accuracy of diagnoses.
Apart from these, clustering and anomaly detection techniques in machine learning also assist in finding patterns, exploring key trends, and identifying anomalies in unstructured datasets, thereby transforming raw data into actionable insights.
Case Studies of Implementations
A variety of healthcare organizations worldwide are already leveraging machine learning to handle unstructured data, converting challenges into tangible health outcomes.
For instance, researchers in various institutions are leveraging AI and machine learning to scan electronic health records and predict outcomes better. John Hopkins researchers, for example, developed an AI analysis yielding personalized predictions of survival in people with cystic fibrosis, by analyzing both structured and unstructured data from electronic health records.
On another front, Mayo Clinic developed an NLP system to process unstructured pathological reports to identify index cancer cases with a high degree of accuracy. This AI-based method saved time for medical coders and reduced manual error, enhancing data availability for cancer research and management.
Moorfields Eye Hospital in London, in collaboration with DeepMind, developed an AI system that analyzes eye scans and makes referrals recommendations, cutting down unnecessary appointments and potentially preventing significant sight loss.
These are just a handful of examples portraying the strength of machine learning in handling and exploiting unstructured data in healthcare. The widespread benefits range from better disease prediction, diagnosis and treatment, improved workflow efficiencies, to advanced research and patient care.
How to Approach Integrating AI and Machine Learning for Unstructured Data in Healthcare
Incorporating AI and machine learning into a healthcare data strategy requires a comprehensive and meticulous approach. A roadmap for effective implementation begins with strategic planning. Understanding the organizational needs, identifying the key areas where machine learning can generate meaningful impacts, and setting realistic goals are foundational steps.
Subsequently, choosing the right tools and partners becomes crucial. The market today is flooded with numerous AI-based tools and platforms, making the selection process a daunting task. A thorough assessment should include an examination of the tool's capacity to handle and process large volumes of healthcare data swiftly and effectively.
In addition, when dealing with sensitive healthcare information, considerations of legal and ethical dimensions are indispensable. Establishing appropriate data governance and conforming to privacy regulations should be inherent in AI strategy. Because patient data security is paramount, platforms ensuring data encryption and providing secure access protocols should be chosen.
Future Potential of Unstructured Data in Healthcare
Peering into the future, the potential of unstructured data in healthcare goes beyond the imaginable. Predictive analytics, powered by machine learning and AI, can revolutionize healthcare, enabling pre-emptive medical interventions and shifting the healthcare paradigm from reactionary to preventive.
Next-generation sequencing and genomic data, a rich source of unstructured data, have immense unexplored potential. As more insights are derived, physicians will have a powerful tool at their disposal, driving precision medicine.
Additionally, the combination of IoT and machine learning can transform unstructured data from health wearables and remote monitoring devices into clinical insights. These wearable IoT devices produce extensive unstructured data that can be harnessed for timely health advice, remote patient monitoring, and improving overall patient engagement.
In summary, the future of unstructured data in healthcare looks promising, potentially imbuing the industry with advanced patient care, improved health outcomes, and proactive disease management.
The Road Ahead for Unstructured Data in Healthcare
With an unprecedented increase in data generation, the criticality of managing unstructured data in healthcare is more relevant than ever. Although challenges exist, the confluence of AI and machine learning technologies presents an innovative solution. NLP, deep learning, clustering and anomaly detection all form an integral part of the toolkit that offers new ways to interpret this data.
The evidence of successful use cases further validates this approach. Institutions worldwide are already witnessing accelerated diagnosis, improved patient care and advanced research capabilities from effective utilization of unstructured data.
However, the journey towards efficient implementation requires a meticulously planned strategy, selection of the right tools, and adherence to key legal and ethical guidelines. With these considerations in place, the path ahead seems promising.
With advancements in predictive analytics, integration of genomic data, and the role of IoT, the potential of unstructured data in healthcare continues to grow. Moving ahead, the focus for healthcare institutions would be to fully exploit these technological advancements to catalyze the shift towards proactive and personalized healthcare.
If you're interested in exploring how Deasie's data governance platform can help your team improve Data Governance, click here to learn more and request a demo.