Tools for Data Governance: Streamlining Your Data Management Process
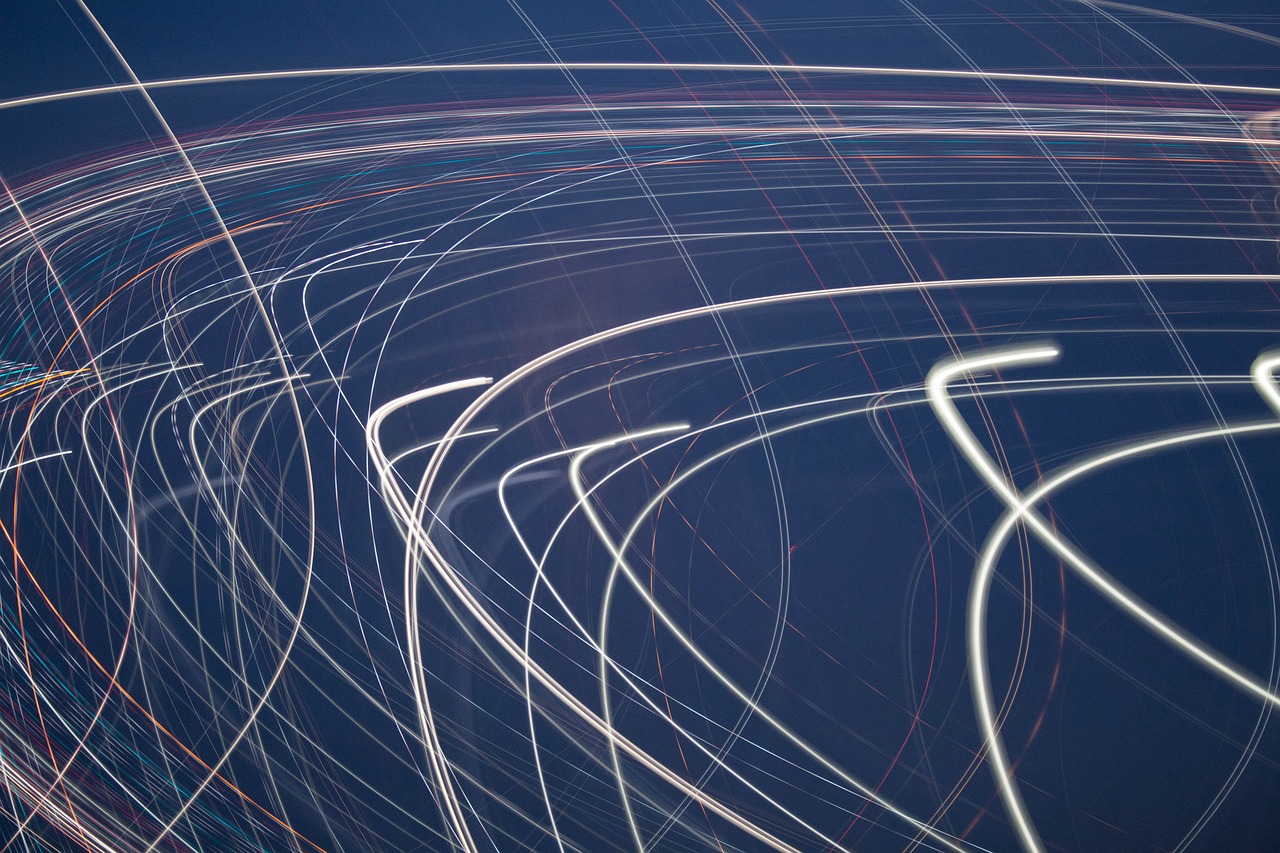
Understanding Data Governance
Data governance is the systematic management of data within an organization. It involves establishing methodologies, processes, and practices to ensure the organization's data is accurate, consistent, secure, and readily available. In essence, it's the framework that provides control over data assets, ensuring that they can be trusted and are accessible to those who need them.
In regulated industries such as finance, healthcare, and government, data governance is of paramount importance. It's not just about maintaining data integrity—it's about regulatory compliance, protecting client confidentiality, and securing sensitive business information. Proper data governance can improve decision-making, mitigate risks and reduce operational inefficiencies. It becomes the backbone of an organization's efforts towards informed business strategies, risk management, and overall customer satisfaction.
Challenges in Data Governance
Implementing an effective data governance framework can be a complex task. To better appreciate the need for robust data governance tools, let's delve into some of the inherent challenges involved.
Data Quality
Poor data quality is a formidable challenge in data governance. This comes in the shape of incomplete data, inaccurate data, or redundancies. Poor data quality can severely impact an organization's operational effectiveness, analytical precision, and decision-making processes.
Data Consistency
Maintaining data consistency across numerous departments and teams is a notable challenge in data governance. In global enterprises, with data originating from diverse sources in varying formats, ensuring data consistency and eliminating discrepancies is a task easier said than done.
Securing Sensitive Data
Securing sensitive data and protecting it against breaches is a fundamental aspect of data governance. As data becomes an increasingly valuable asset, the risk of cyber threats and hacks increases parallelly. Developing robust data security protocols is therefore a critical part of data governance.
Complying with Regulations
Regulated industries are under constant pressure to stay compliant with evolving regulations. Issues around data privacy such as GDPR, CCPA, and HIPAA require stringent data governance policies. Non-compliance can lead to penalties, tarnish brand reputation, and shake customer trust.
Navigating through these challenges necessitates robust tools and practices for data governance. By understanding these challenges, organizations can better design or select tools to mitigate these obstacles and streamline their data management process. Better articulation of data governance policies, implementing effective tools, and encouraging wider participation across the organization are steps towards overcoming these hurdles.
Essential Components of Data Governance
Let's break down data governance into its essential components. These are key areas where the right tools and systems can make a marked difference in an organization's data management strategy.
Data Quality Management
Data quality encompasses completeness, consistency, and accuracy. Great governance requires tools that can automate data cleansing, verify and maintain data accuracy, validate data consistency, and ensure data completeness. Without reliable data, decision-making processes and regulatory compliance become difficult.
Metadata Management
Metadata is data about data. It provides crucial insight into the content, context, and source of data. A robust metadata management tool helps enterprises understand data better, trace data origins, and establish strong search and indexing capabilities. This facilitates quicker decision-making and helps resolve data issues more effectively.
Data Security Management
This involves tools that can secure sensitive data from internal and external threats. Segregating data based on roles, enforcing data access controls, and deploying data encryption are some of the steps that can enhance your data's protection. Without a doubt, safeguarding business-critical data is fundamental to sound data governance.
Database Management
Tools that can create, read, update, and manage databases effectively form the cornerstone of good data governance. Database Management Systems (DBMS) serve this purpose, facilitating better data storage, retrieval, modification, and deletion processes. The type of DBMS to adopt—be it Relational DBMS, NoSQL DBMS, or others—depends on the organization's specific business needs and data types.
Compliance Management
Depending upon the industry and geographical presence, compliance regulations can be complex and numerous. Compliance management tools help organizations stay ahead of the curve by identifying potential areas of non-compliance, automatically updating changes in relevant laws, and generating compliance reports. It’s a way of ensuring you’re ticking all regulatory boxes.
Tools for Streamlining Your Data Governance Process
Certain tools have been proven to streamline the data governance process significantly. Let's examine them in more detail.
Master Data Management (MDM) Tools
MDM tools focus primarily on providing a single, consistent, and accurate source of business-critical data—also known as master data—across the enterprise. They aid in avoiding data duplication, ensuring data consistency, and establishing cross-linkages between disparate data sources. Example MDM tools include Informatica MDM, SAP NetWeaver, and IBM InfoSphere.
Data Catalog Tools
Data Catalog Tools generate a centralized inventory of data sources, making data discovery more straightforward for users. By providing users with a clear view of available data, its location, and its relevance, these tools foster better data comprehension and usage. Examples are Google Cloud Data Catalog, Alation, and IBM Watson Knowledge Catalog.
Data Quality Tools
Companies use tools to evaluate, monitor, and enhance the quality of data. They help in identifying dirty data, running data profiling, automating data cleaning, and validating data post-cleansing. Prominent examples are Informatica Data Quality, Talend Data Quality, and IBM InfoSphere QualityStage.
Data Protection Tools
Organizations use data protection tools to secure sensitive data from leaks, thefts, and breaches. They aid in deploying encryption protocols, establishing role-based access, and enforcing data integrity checks. Some examples encompass Symantec Data Loss Prevention, Digital Guardian, and McAfee Total Protection for Data Loss Prevention.
Adoption and successful implementation of these tools significantly alleviate challenges seen in data governance. They not only enhance the robustness of business data but also pave the way for other advanced, AI-based data governance measures.
How Machine Learning and Artificial Intelligence Enhance Data Governance
Cutting-edge technologies like Machine Learning and Artificial Intelligence play an increasingly crucial role in modern data governance, providing solutions that go beyond traditional methods.
Machine Learning in Data Quality
Machine learning algorithms can process and analyze vast volumes of unstructured data in real time. They aid in identifying patterns, detecting anomalies, and predicting trends – providing deeper insights into data quality. From improving data acquisition to refining cleansing methodologies, machine learning transforms the way organizations ensure the quality of their data.
AI in Data Compliance
Artificial Intelligence is making waves in the realm of data compliance. AI-powered systems can analyze different regulatory texts, extract obligations, and map them against organizational policies. This rapid, automated assessment considerably reduces the risk of non-compliance. As regulations grow more complex and diversified, AI offers a scalable solution for meeting compliance challenges.
Predictive Analytics in Governance
Predictive analytics combines statistical analysis, machine learning, and data mining to predict future events. It can streamline data maintenance by predicting data errors or inconsistencies before they occur. This proactive approach leads to more efficient data correction and prevention strategies, substantially improving the overall data governance framework.
Case Study: Successful Implementation of Data Governance Tools in Enterprise
Let's take a look at a real-world example of how proper tool implementation enhances data governance.
An international bank with diverse operations and a large customer base faced challenges with data inconsistency, resulting in impaired decision-making and regulatory violations. At the core of these issues was the lack of a consolidated, consistent view of customer data scattered across regions and departments.
The bank decided to implement a Master Data Management (MDM) tool to create a 'single version of truth' for its customer data. They also adopted a data quality tool to continuously cleanse, monitor and validate data. To maintain regulatory compliance, they executed a compliance tool that mapped the bank’s policies against rapidly changing financial regulations.
Post-implementation, the bank witnessed significant improvements. The MDM tool helped to standardize, de-duplicate, and create an accurate customer database. The data quality tool rectified legacy data issues and established robust data quality checks. The compliance tool simplified the process of staying abreast of regulatory changes and ensured their policies met all requirements.
Real-world examples like this drive home the transformative potential of data governance tools in large enterprises. The benefits of such tools are tangible and significant, contributing to more robust data management processes and better organizational outcomes.
Future of Data Governance: New Trends and Innovations
Data governance isn't static—it evolves constantly to keep pace with dynamic business climates, technology advancements and regulatory modifications. Let us explore the future prospects of data governance:
Increased Adoption of AI & ML in Governance
The promise of Artificial Intelligence and Machine Learning in Data Governance is gearing up to reach its full potential. As seen previously, AI and Machine Learning open avenues for automated data governance, real-time data quality issue detection, predictive data maintenance, and more. This trend seems poised to flourish in the coming years as more companies realize its potential.
Rise of DataOps
DataOps, or Data Operations, is an agile methodology designed to improve the speed, quality, and reliability of data analytics. It enhances collaboration, automates data pipelines, and uses advanced tools to provide precise, real-time insights. Incorporating DataOps into data governance can empower organizations to reduce cycle time, maintain high data accuracy, and increase the confidence of business stakeholders in the data.
Stringent Data Privacy Laws
The focus on data privacy is growing exponentially, resulting in more rigorous, consumer-centric laws worldwide. These laws demand greater transparency, informed consent, data minimization, and breach notifications. Organizations will have to adapt to these changes and ensure their data governance strategies are in alignment with such laws.
The future of data governance seems dynamic, challenging, and exciting. The rise in innovation, coupled with increasingly stringent data regulations, suggests a future where data governance will be a central part of business strategy and decision-making.
If you're interested in exploring how Deasie's data governance platform can help your team improve Data Governance, click here to learn more and request a demo.