Managing Metadata: Best Practices for Efficient Data Categorization
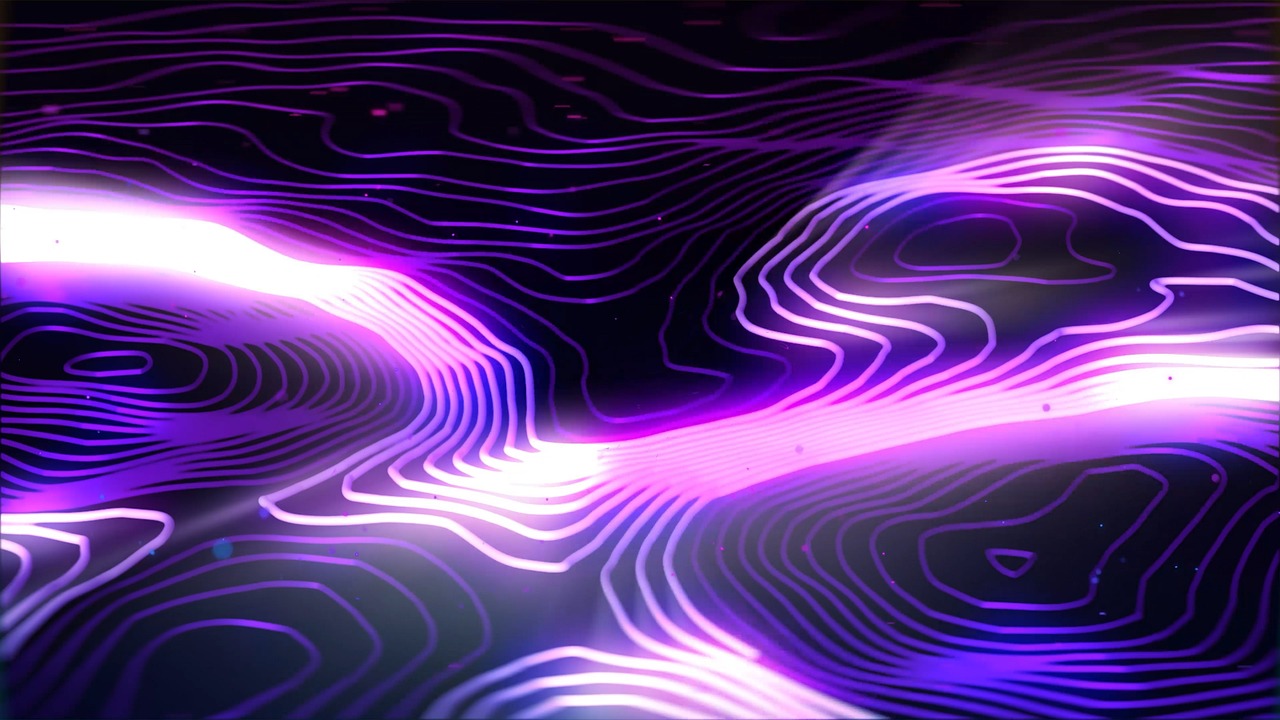
Understanding Metadata: An Overview
Metadata: the word may not carry the glamor associated with terms such as machine learning or artificial intelligence, but it shares an equal, if not higher, degree of importance in the realm of data management. Meta, a prefix from the Greek μετα-, means "after" or "beyond". When combined with "data," it signifies something that resides beyond the data. In other words, metadata is data about data.
In the vast universe of digital records, think of metadata as the stars helping you navigate unknown waters. It provides a comprehensive summary and a contextual framework to understand complex databases. It spells out what the data represents, how it interrelates, and provides meaningful insight into the content, quality, condition, origin, and location of data resources. For instance, in a document file, metadata might include details such as the author's name, date of creation, file size, or the date it was last modified.
Without metadata, sorting through the colossal heaps of data, making decisions, or even planning interventions at enterprise-level operations would be a substantial challenge. It forms the backbone of a myriad of processes, from the complex workings of search engines and content personalization to data governance in regulated industries.
Types of Metadata
Delving deeper into the milieu of metadata, it primarily exists in three forms: Descriptive, Structural, and Administrative. Each of these types plays a unique role, thereby providing multiple layers of insight into the underlying data.
- Descriptive Metadata: This type of metadata hinges largely on identifying and discovering resources. It involves information such as title, summary, authors or creators, keywords, and more. In a library, for example, details like the ISBN number, book title, author, publisher, and publication date form descriptive metadata. It essentially creates a foundation for resource discovery, aiding users to search, identify, and pull out relevant resources.
- Structural Metadata: As the name suggests, this form provides a layout, depicting how compound objects are put together, for instance, how pages are ordered to form chapters. Structural metadata simplifies navigation through resource components, making it indispensable for digitized content management.
- Administrative Metadata: This category emphasizes the management aspect of digital resources. It includes details that help manage a resource, like creation date, file type, and permissions. Administrative metadata aids in maintaining the lifecycle of a resource, slated for preservation, rights management, and assessment.
Together, these types contribute a comprehensive understanding of a data resource. Managing metadata effectively can exponentially improve the accessibility, traceability, and usability of data across a wide array of applications.
The Role of Metadata in Machine Learning and AI
Make way for the unsung hero of Machine Learning (ML) and Artificial Intelligence (AI): Metadata. In an AI-driven world, data is the key driver, and metadata takes the role of a proficient backseat driver. It steers the technology through AI model lifecycle - from understanding the data and fine-tuning models to the management of AI models.
In the data understanding phase, metadata becomes indispensable. It provides clarity on a data set’s attributes, structure, and relations, propelling insightful decisions for data preprocessing and model selections. This metadata-powered understanding is crucial when dealing with complex, multi-dimensional, and real-world data.
During model training and validation, metadata of the hyperparameters, training runs, and experimental features helps monitor the process, compare models, and reproduce results, thus fueling ML model explainability. It also aids in investigating bias and fairness in AI.
As enterprises scale their AI deployments, managing AI models becomes critical. Metadata comes to the rescue by tracking models' performance, identifying when models drift from their original accuracy, and signaling necessary maintenance or update. By ensuring traceability and transpareteness of AI models, metadata forms the cornerstone of AI Governance and mitigates potential risks.
Challenges in Managing Metadata
Despite its significance, managing metadata isn't a breezy affair. When it comes to obtaining the full benefits of efficient metadata management, companies grapple with several challenges.
One such challenge revolves around handling the colossal volume of metadata churned out by daily business processes and digital interactions. Since the amount of digital data generation is increasingly exponential, managing this vast landscape of metadata requires advanced tools and strategies to avoid being overwhelmed.
Next, maintaining data consistency also proves to be a tough row to hoe. With disparate data sources and diverse data standards, ensuring that metadata remains consistent across systems is daunting. A discrepancy in metadata could lead to flawed data sets, thereby misleading insights and decisions.
Finally, data security and privacy become crucial areas of concern in metadata management. With stricter regulations and increased awareness about data privacy and protection, securing metadata from potential breaches while adhering to the regulatory compliance has become imperative.
Successfully maneuvering through these obstacles requires a structured approach to metadata management - from employing best practices in data governance to the deployment of effective metadata management tools. By addressing these challenges head-on, enterprises can make strides toward leveraging metadata to its maximum potential, fostering a truly data-driven culture.
Key Strategies for Efficient Metadata Management
With digital innovation skyrocketing, data management is no longer optional. Metadata management, in particular, holds a paramount place in business processes in achieving effective business analytics, regulatory compliance, and data lineage. A sound metadata management framework integrates three pivotal elements: robust data governance, a hierarchical approach to metadata categorization, and the use of metadata management tools.
Data governance has a significant role as it preserves the consistency, clarity, and reliability of the metadata. Effective data governance sets the stage for policies and procedures that ensure high metadata quality across an organization — thus optimizing data reliability, streamlining operations, and promoting efficiency.
Meanwhile, deploying a hierarchical approach to metadata categorization, wherein metadata is structured based on a hierarchy of categories and subcategories, boosts searchability and navigability, especially in massive data pools. This hierarchical organization brings an order to chaos, making it easier to locate, understand, and use the data efficiently.
Last but not least, employing metadata management tools can automate the often labor-intensive process of metadata collection, validation, and updating. These tools facilitate efficient handling and utilization of metadata, reducing the risk of errors and inconsistencies.
Best Practices for Metadata Categorization
With an established strategic framework in place, it becomes pivotal to honor best practices for metadata categorization. These practices are designed to ensure the optimization of metadata, thereby enhancing its utility and facilitating efficient data management.
A standout best practice is the standardization of metadata descriptions. It is essential for businesses to establish a standard set of terms that eliminates ambiguities and promotes a clear understanding of the metadata. This practice not only simplifies data usage but also promotes uniformity across various workgroups within the organization.
Another key aspect involves conducting regular metadata audits. These systematic examinations can ensure metadata consistency and accuracy, identifying any discrepancies or gaps in the metadata. Regular audits keep the metadata up-to-date and in synchronization with the evolving business processes and systems.
Furthermore, encouraging 'meta-tagging', where data or content is tagged with relevant metadata, can enhance the visibility of your data. Such metadata tags make data more searchable and accessible, helping users find the information they need more efficiently.
Moving beyond these best practices, emerging technologies like AI have begun to revolutionize metadata management. Leveraging these advancements can empower enterprises to manage metadata more resourcefully, extracting greater insights and value from their data resources.
The Role of AI in Metadata Management
Positioning itself as the North Star for digital innovation, Artificial Intelligence has infiltrated the sphere of metadata management, ushering in a new era of efficiency and foresight. Though still in infancy, AI-driven metadata management approaches hold immense promise for navigating the future of data-driven decision making.
When AI meets metadata management, we see the birth of automated metadata categorization. By deploying AI algorithms, tedious manual processes of metadata collection, tagging and categorization can be automated, guaranteeing accuracy and saving valuable time. Furthermore, by integrating machine learning for the automatic detection and tagging of metadata, organizations drive higher productivity levels, enabling users to retrieve and utilize data with unprecedented ease.
Progressing a step further, AI introduces the concept of predictive metadata analysis. Through advanced analytics, AI can predict trends, detect anomalies, and offer insights on large metadata sets. This paves the way for a more strategic use of metadata, where organizations can perform preventative maintenance and take timely action based on predictive outputs.
Another significant application of AI lies in enhancing metadata security. By employing AI algorithms for anomaly detection, potential breaches can be identified and prevented, strengthening metadata security infrastructure.
Case Study: Metadata Management in Regulated Industries
Regulated industries such as financial services, healthcare, and government often deal with massive volumes of sensitive data, necessitating a robust metadata management practice.
In financial services, organizations navigate a sea of transactions, customer information, and financial records. Metadata management assists in understanding this data labyrinth, achieving compliance, detecting fraudulent behavior, and driving strategic decision-making. With an effective metadata management regimen, financial institutions can enhance risk management, ensure regulatory compliance, derive valuable insights, and enhance customer experience.
Similarly, the healthcare industry generates copious amounts of patient data, research findings, treatment records, and more, making efficient metadata management invaluable. Metadata in healthcare guides professionals in making sense of complex patient data, improving patient care, streamlining research processes, and aiding in achieving compliance with laws like HIPAA.
Lastly, the government sector, known for its multifaceted data landscape, ranging from citizen data to confidential state affairs, can leverage effective metadata management. By enhancing data accessibility, facilitating efficient information retrieval, and ensuring stringent regulatory compliance, metadata management aids government bodies in delivering better public services and maintaining the trust of citizens.
By encapsulating these real-world applications, we comprehend the critical necessity of efficient metadata management paradigms in regulated industries, and the growth potential it offers for the future.
If you're interested in exploring how Deasie's data governance platform can help your team improve Data Governance, click here to learn more and request a demo.