Framework for Data Governance: Designing the Roadmap to Data Mastery
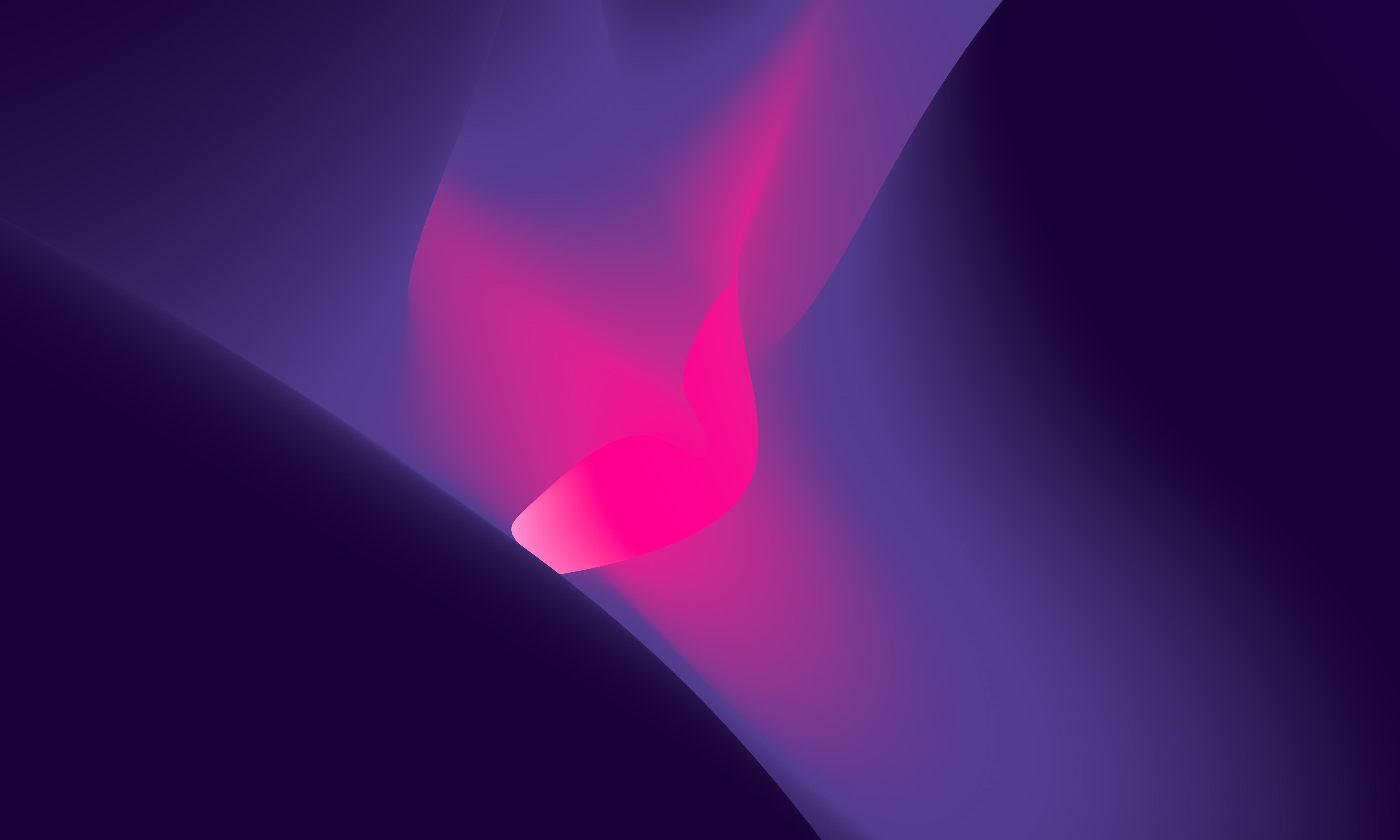
Understanding Data Governance
In the era of big data and with the continuous infusion of artificial intelligence and Machine Learning technologies into the business spectrum, Data Governance has evolved from being just a mere concept to an integral organizational strategy. Data Governance is a holistic approach to managing, improving, and leveraging data to make business decisions, reduce operational friction, protect the business, and enhance service delivery.
At its crux, Data Governance accentuates the overarching management of the availability, usability, integrity, data quality, and security of enterprise data. It involves setting key strategies and tactics around data handling, establishing data standards and policies, and implementing practices to driving organizational adherence to optimize data quality.
The significance of Data Governance lies not only in its role as a protective shield to ensure data privacy and compliance with various laws and regulations, but it's also a catalyst fostering data quality and efficacy - imperative for crisp data-driven decisions. This, in turn, amplifies the ability of a business to meet its objectives, maximize profits, and retain a competitive edge. In a landscape where data is the new oil, a robust Data Governance strategy is instrumental in distilling this oil to fuel the engines of business growth.
The key components of a stout Data Governance framework encompass the trifecta of people, processes, and technology. Success in Data Governance arises from judiciously integrating these elements - setting a clear sense of accountability and ownership with the people handling data, standardizing the processes around data lifecycle management, and employing advanced technology aid to streamline these processes.
Nevertheless, each organization is unique, with distinctive business needs and objectives. A one-size-fits-all approach to Data Governance is but a myth. Designing a Data Governance framework demands a keen understanding of the unique needs of the business, aligning the governance initiatives with these needs, and creating a customized roadmap for implementation. This roadmap, acting as a compass, guides the organization on the path to achieving data mastery. With the burgeoning volumes of data in the modern digital era, mastering the art of steering the data ship with adept Data Governance is no longer a mere luxury, but the need of the hour.
Planning Your Data Governance Journey
Nailing the right Data Governance strategy is contingent upon an astute understanding of the organization's data needs and the well-defined goals expected to be achieved through a robust Data Governance framework. The distinctive characteristic of every organization necessitates a unique approach to Data Governance, guiding a tailored journey on the path to data mastery.
The first stride on this path is to gauge the organization's data needs. A thorough audit of the existing data assets should be conducted to comprehend the data landscape - understanding its origin, nature, and the scope of its usage across various verticals in the organization. This will aid in identifying the strengths and potential areas of improvement in data management, thereby, providing a tangible insight into the necessary measures required uplift the existing data practices.
The next step in charting out the Data Governance journey is defining crisp, realistic, and measurable goals. These objectives should resonate with the broader business strategy, aimed at uplifting the overall organizational performance. Effective data governance might manifest itself in diverse forms - ranging from elevating data quality, ensuring data consistency across business units, enhancing regulatory compliance, to improving data security, among others. Having clarity on these goals will aid in identifying the apt governance policies and rules to be instated, keeping the business objectives at the forefront.
Identifying the key stakeholders in data governance is a critical aspect of planning the governance journey. These stakeholders encompass data owners, stewards, users, and custodians, each vested with different responsibilities in the data lifecycle. Formally acknowledging these roles within the organization fosters accountability and emphasizes the gravity of maintaining high data integrity and quality.
For instance, a data owner would typically be a high-ranking executive with the onus of policy setting and ensuring compliance with data regulations. A data steward, on the other hand, would be responsible for the enforcement of these policies and managing the quality of data input and output. Recognizing these roles is vital in clearly delineating responsibilities and creating a strong governance structure.
Implementing a Framework for Data Governance
Unfurling an effective Data Governance framework is a meticulous process that calls for strategic thinking and shrewd execution. The linchpin of this progression lies in aptly structuring data, ensuring the sanctity of data, safeguarding data privacy, and setting in place effective data retention policies.
A well-knit data governance strategy hinges on efficient data categorization and sturdy organizational hierarchies. The stratagem behind this lies in framing a logical structure that binds together data assets and thereby fostering ease of access and usage. Instituting a data catalog serves this purpose by enabling systematic organization and classification of data, which in turn accentuates tighter governance. A data catalog suffused with metadata detailed annotations points towards a holistic, well-structured view of the organizational data that paves the path to firmer data governance.
As crucial as streamlined data management is, the vitality of ensuring data quality cannot be undermined. From an organizational perspective, high-quality data is indicative of comprehensive, accurate, and relevant information that is expedient in decision-making processing. To effectively uphold this, the implementation of data governance should entail mechanisms to identify and rectify data discrepancies and inaccuracies, ensuring the data is authentic, reliable, and primed for utilization.
In an age where data breaches are rampant, firms are tightening their grip on data security to ward off potential threats. Implementing a data governance framework requires putting in place robust data protection measures aligned to meet the mandatory compliance requirements and regulations. It's pivotal to instate stringent controls on data access, regularly monitor and audit data usage, and vigilantly guard against data thefts to navigate the turbulent seas of data security.
An often overlooked yet crucial aspect of data governance is establishing effective data archiving and retention policies. These dictate the stipulations around data storage, retention schedules, archival processes, and data destruction protocols after it's no longer required. A cogent policy aids in managing data efficiently, complying with regulatory requirements, and reducing data storage costs.
Effectuating a Data Governance framework thus involves a delicate orchestration between strategic data organization, quality control, stringent security measures, and effective data retention policies. Navigating this complex maze successfully will draw the line between rudimentary data management practices and a robust Data Governance framework, steering the organization towards the ultimate goal of data mastery. This process, though intricate and time-consuming, is a worthy investment that will pay rich dividends in terms of business efficiency and growth in the long run.
Role of Machine Learning and AI in Data Governance
Machine Learning and AI have risen as game-changers in this increasingly data dominated business realm. As much as businesses leverage these technologies to understand their customers and make strategic decisions, these innovative tools have emerged as potent forces to revolutionize Data Governance strategies.
One of the significant contributions that AI has made in the sphere of Data Governance is the automation of data classification and taxonomy. Classification refers to the method of categorizing data based on its type, contents, and other features. Conventionally, this process has been manual and recursive, calling for a considerable expenditure of time and resources. With machine learning algorithms, this process can now achieve a level of automation. By training models on pre-classified datasets, they can efficiently label and categorize new data, thereby, streamlining data organization and aiding in more effective governance.
Fixating on maintaining data quality is of paramount importance in Data Governance, and this is precisely where AI can render an impactful contribution. AI algorithms can be deployed to detect irregularities and discrepancies and highlight potential errors in data. Furthermore, by learning from the corrections made, these AI systems can predict and rectify such errors in the future, thereby leading to improvements in overall data quality.
Predictive Analytics, powered by machine learning, opens new dimensions in Data Governance. These sophisticated algorithms can analyze current and historical data to predict future trends, thereby providing valuable insights and foresight into potential data growth or data processing needs. This tech-driven insight drastically bolsters the decision-making process and amalgamates greater efficiency into the Data Governance framework.
Measuring Success in Your Data Governance Framework
Quantifying the efficacy of the Data Governance framework forms the cornerstone of its continuing evolution and refinement. The measure of success in Data Governance lies in the translation of its objectives into tangible improvements in business operations, strategic decision-making, and regulatory compliance.
A pivotal element in this quest to evaluate the effectiveness of Data Governance is the identification of key performance indicators (KPIs). These pointers provide quantifiable metrics which could span across different parameters such as data quality, data usability, data compliance, and data protection. Each organization, depending on the particulars of its goals and needs, sets forth its unique KPIs to gauge the progress and effectiveness of its data governance operations.
Quantifying and tracking these KPIs over time provides a clear insight into the performing elements and potential areas of improvement. For instance, a data quality KPI could be the percentage of data errors identified and corrected. Noticing a decreasing trend in this KPI over time would signal an improvement in data accuracy and integrity.
While laying out these assessment metrics, it's essential to ensure that these measures are closely aligned with the organization’s operational objectives. The goal is to ensure that each KPI ties back to a business outcome – be it revenue growth, risk mitigation, efficiency improvements, or customer satisfaction.
Evaluating the Data Governance framework against these KPIs would subsequently expose the tangible benefits that the organization yields - in cost savings owing to improved data quality, increased revenue contributions due to better data-driven decisions, improved regulatory compliance, or enhanced customer satisfaction.
The journey of Data Governance does not reach its destination with the establishment of a framework. Its essence lies in the constant tweaking and calibrating of the strategies based on the KPIs and the insights derived from them. This iterative process of assessment and refinement forms the crux of an effective and sustainable Data Governance strategy - one that evolves and adapts with the changing needs of the business landscape.
Case Study: Data Governance in Action
Delving into a real-life instance of effective Data Governance, let's consider the case of a leading global financial firm, which embarked on the journey of Data Governance to mitigate data-related risks, ensure compliance with prevalent regulations, and improve business performance.
The organization, with a presence in more than 50 global markets, grappled with vast amounts of data spread across various divisions. Handling this massive data pool was challenging, and inconsistencies in data management spanned across the board. Errors and inaccuracies in data, coupled with inefficient processes and regulatory compliance requirements, necessitated a robust Data Governance program.
The firm initiated its Data Governance journey by identifying its data needs and setting clear goals – enhancing data conformity across divisions, elevating data quality, and solidifying regulatory compliance. The steering committee included representatives from all business areas to ensure a broad perspective on data needs and to foster accountability and ownership for maintaining data quality.
The implementation journey included creating a data dictionary to promote a common understanding and consistency of data across the firm. Practice of metadata management, data quality initiatives like automated error detection and correction mechanisms, and stringent data security measures were introduced. An advanced data lineage tool was employed to track data throughout its lifecycle, crucial for detecting errors and demonstrating regulatory compliance.
An AI-based technology solution was adopted to automate the data classification process and implement predictive analytics for forecasting future data needs. Threading together these multiple facets of Data Governance led to the establishment of a firm-wide governance infrastructure that enhanced data usability, reliability and security.
The firm's governance efforts paid off, with concrete improvements observable in business operations. Data errors dropped by a significant degree, regulatory compliance showed an uptick, and data-driven decision making led to improved business strategies. This holistic approach to Data Governance fortified the firm's data framework, and the measurable benefits accrued validated the effectiveness of their Data Governance program.
The Future of Data Governance
With the proliferation of data and technology, the future of Data Governance is set to touch new horizons. Cloud computing has emerged as a significant player in reshaping data storage and management strategies. The allure of cloud storage lies in its promise of scalability, seamless accessibility, and cost-effectiveness. Adapting Data Governance to this new paradigm calls for the establishment of secure cloud policies, robust data privacy controls and reliable data recovery mechanisms. Data Governance in a cloud environment should ensure accurate labeling, track data lineage and monitor data movement to maintain data integrity, all while complying with regulations specific to cross-border data transfers and privacy.
Moreover, technological advancements like 5G promise more efficient data transmission, with increased capacity and lower latency. Leveraging the speed and reliability of 5G networks can fundamentally transform data-related operations, including real-time analytics, IoT communication, edge computing, and more. With these advancements comes the challenge of managing and securing an even larger volume of data. The Data Governance of tomorrow will have to grapple with these challenges, ensuring that data quality, privacy, and compliance are not compromised amidst the burgeoning data volumes.
The key to future-proof Data Governance lies in embracing these technological advancements, adopting agile governance frameworks, and instilling a culture of continuous data improvement. Constructing adaptive governance policies, harnessing AI for automated data management, and committing to ongoing training and awareness for stakeholders are key steps in this direction.
The future of Data Governance is a landscape of opportunities waiting to be seized. With the right strategies, cutting-edge technology, and an organizational commitment to data quality, businesses can cultivate a future-ready Data Governance Framework that is primed to harness the potential of the data-rich world of tomorrow.
If you're interested in exploring how Deasie's data governance platform can help your team improve Data Governance, click here to learn more and request a demo.