Data Quality Tool: The Solution for Enhanced Data Reliability and Accuracy
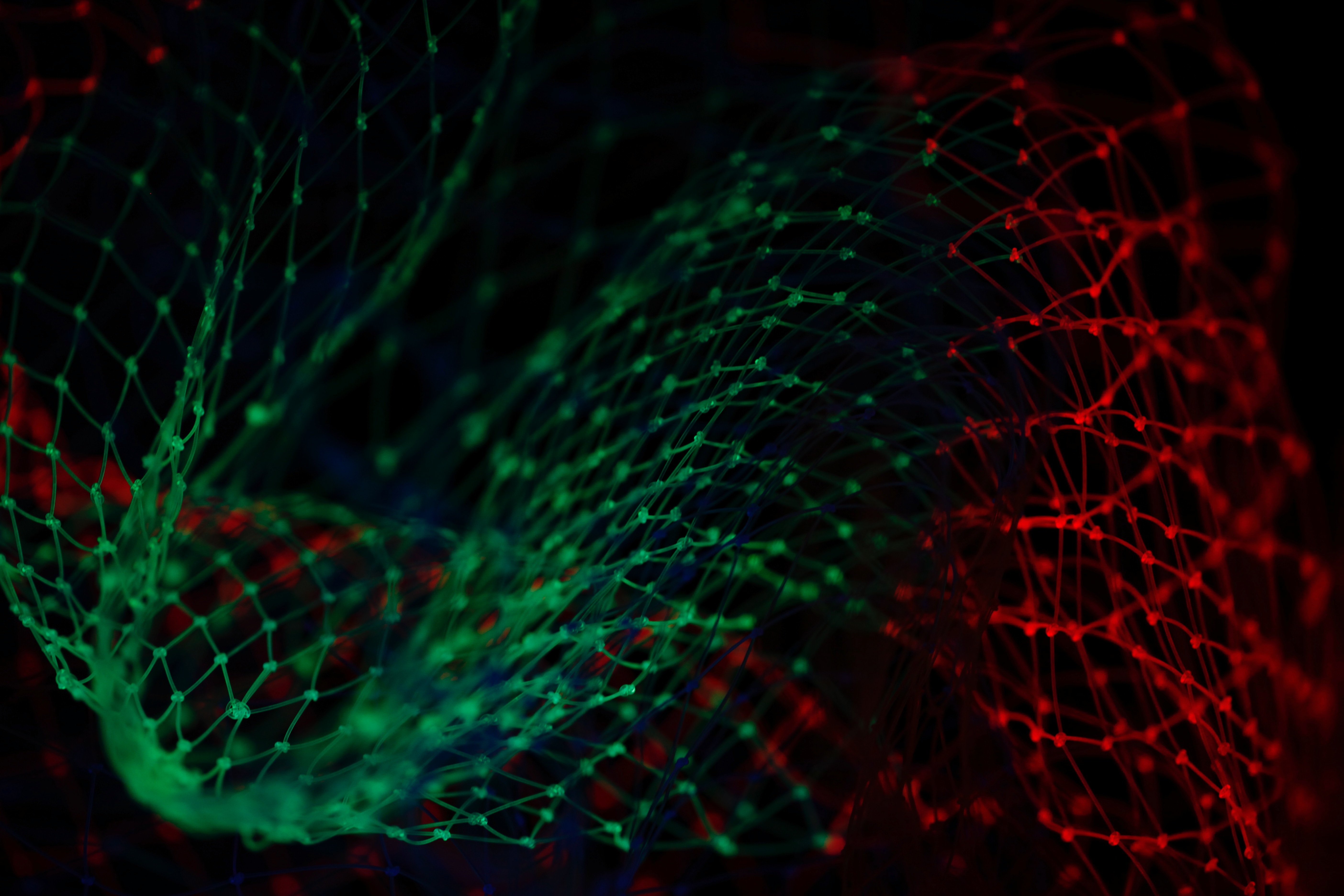
Importance of Data Quality
In the burgeoning realm of digitalization, data has emerged as a strategic asset for enterprises, fueling key decisions, enhancing customer relations, and sculpting competitive differentiators. One of the challenging facets of managing this asset is ensuring its quality. The reliability and accuracy of data are vital as inaccuracies can lead to misguided strategies, poor customer service, and compliance liabilities.
Quantifying the cost of poor data quality unearths serious implications. Erroneous data can cause wrong predictions, compromise strategic decision-making, and lead to operational inefficiencies. In industries where regulation is stringent, such as finance and healthcare, the repercussions can reach as far as hefty fines and reputational damage.
On the flip side, reliable and accurate data breeds a myriad of benefits. Accurate data feeds into precise analytics and sound business intelligence, guides effective decision-making, streamlines operational processes, and ultimately culminates in improved bottom-line outcomes. The key to unlocking these benefits lies in leveraging the right data quality tools.
The Role of Data Quality Tools
Data quality tools serve as a linchpin in managing the accuracy and reliability of data. These are specialized software applications that help ensure that data sets are error-free, consistent, and usable for analysis or business operations.
Data Validation Tools
These tools confirm the validity and consistency of data according to the pre-defined business rules or database regulations. They play a crucial role in ensuring data integrity, a fundamental requisite of high-quality data.
Data Transformation Tools
Data often needs to be transformed and formatted to be used effectively, especially when integrating data from different systems or sources. Data transformation tools aid in changing the data format, structure, or values to meet analytical or operational requirements.
Data Profiling and Monitoring Tools
Aura of transparency and trust in data's health is crucial for enterprises managing vast amounts of data. Data profiling and monitoring tools come into play here, providing a detailed view of data's attributes, quality levels, and identifying issues before they snowball into more significant problems.
Acknowledge these tools' potency; the expedition to improved data quality becomes a surmountable challenge. But understanding is one thing, choosing the suitable tool quite another, leading us to explore some key data quality tools.
Exploring Key Data Quality Tools
Navigating the data quality landscape involves discerning the offerings of various tools and aligning them with your business requirements. Let's delve into some of these significant players.
Trifacta
Emerging out of the realm of data wrangling, Trifacta is a tool designed to cleanse and transform data for analysis. It offers robust capabilities to navigate through large volumes of data, intelligently spot inaccuracies, and automate the data transformation process.
Talend
Bringing a deep integration capability, Talend serves as the linchpin to unifying disparate data sources. It helps businesses cleanse, match, and transform data to enhance accuracy. It amplifies data quality routines with machine learning and cross-enterprise data governance.
Informatica
Informatica provides a holistic suite of data quality tools. It enables businesses to cleanse, standardize, and enrich enterprise data, and validate consistency across different formats and systems. Its scalable architecture allows it to handle data quality issues across any platform and any data.
Oracle Data Quality
A comprehensive suite catering to data profiling, cleansing, and monitoring, Oracle Data Quality gives an organization the tools to ensure their data's integrity. It shines out with attributes of scalability, high-performance, and enhanced customization, ergo being highly suited to large enterprises having copious data reserves.
Deasie
Deasie carves its unique niche by bringing sophisticated machine learning algorithms to bear on data profiling. As a decentralized AI ecosystem, it makes data quality management efficient and robust, attributable to its predictive analysis and automation capabilities.
Integration of Machine Learning & AI in Data Quality Tools
With the infusion of machine learning and artificial intelligence, data quality tools are on an overdrive to new capabilities and efficiencies.
A significant impact of the AI revolution is the automation of data cleaning. Intelligent algorithms can trawl through monumental datasets, making sense of anomalies and errors, and rectifying them. This aspect has considerable time-efficiency implications, reducing the manual labor and human-induced errors that typically haunt traditional data cleaning methods.
Contributing to the arsenal of AI for data quality, machine learning enhances the predictability of data quality. Machine learning models thrive on vast volumes of data, pulling out patterns, behaviors, and insights. Trained on high-quality data, these models can predict the health and the quality of new data, making for proactive stances in data quality management.
AI and ML aren't just theoretical spectrums of discussions; their practical implications in improving data quality have been inspiring. In complex regulated industries like healthcare, machine learning helps predict patient outcomes, while in the financial sector, it aids in fraud detection.
Moving the center of the discussion to large language models (LLMs) presents another dimension to the use of advanced technologies in refining data quality. Akin to how AI and ML have been disruptive, LLMs carry substantial potentials in galvanizing the quality of data.
The Impact of LLMs on Data Quality Improvement
Veering towards the impressive capabilities of Large Language Models (LLMs), such as GPT-3, they unfold a new epoch in the landscape of data quality. Notwithstanding the complexity of the dataset and language patterns, LLMs are equipped to handle data quality issues uniquely and effectively.
At a fundamental level, LLMs revolutionize the data cleaning process. They provide intuitive grammar corrections, text normalization, and counter duplicity issues in the data. Embedding these models into your workflow ensures higher data quality with a remarkable reduction in manual labor and errors, which are otherwise inevitable in traditional data cleaning.
Adding to their discerning capabilities, LLMs have shown to predict clean and high-quality data. Fortified by the properties of context understanding and the ability to learn from patterns, LLMs navigate through vast volumes of data, predicting accurately, and offering high-quality data.
Moving over to automation, LLMs enable continual data quality monitoring. Their ability to learn from errors, improve over time, and adapt to different patterns, helps them efficiently spot anomalies, thereby, assuring the requisite data quality in a cyclic, constant manner.
Future Prospects for Data Quality Tools
As we navigate the horizon of data quality, emerging technologies and trends perceive an evolution of data quality tools.
Predictive analytics is gaining momentum in the field of data quality. It uses statistical and machine-learning algorithms to make forward-looking predictions based on existing and historical data. The implication in terms of data quality is explicit - businesses could predict potential data quality issues before they inflict operational impacts.
Shifting the attention towards Natural Language Processing (NLP), it has the potential to carve distinct improvements in data quality. Its ability to decipher, interpret, and generate human language resonates well with dealing with unstructured data. It can distill useful information from such data and improve its quality, thus, facilitating robust data analysis.
Taking a delve into Data as a Service (DaaS), it brings forth a new paradigm in data quality. By availing cleaned, processed, and quality-compliant data delivered by DaaS providers, businesses can focus solely on leveraging this data for intelligence, prescriptive analytics, or AI model training.
With the right assimilation of technology and tools, businesses can tackle the challenges of data quality head-on. This ensures they extract the best possible intelligence and insights from their data, empowering them to make informed and strategic decisions.
If you're interested in exploring how Deasie's data governance platform can help your team improve Data Governance, click here to learn more and request a demo.