Data Quality Management: Ensuring Accuracy and Integrity
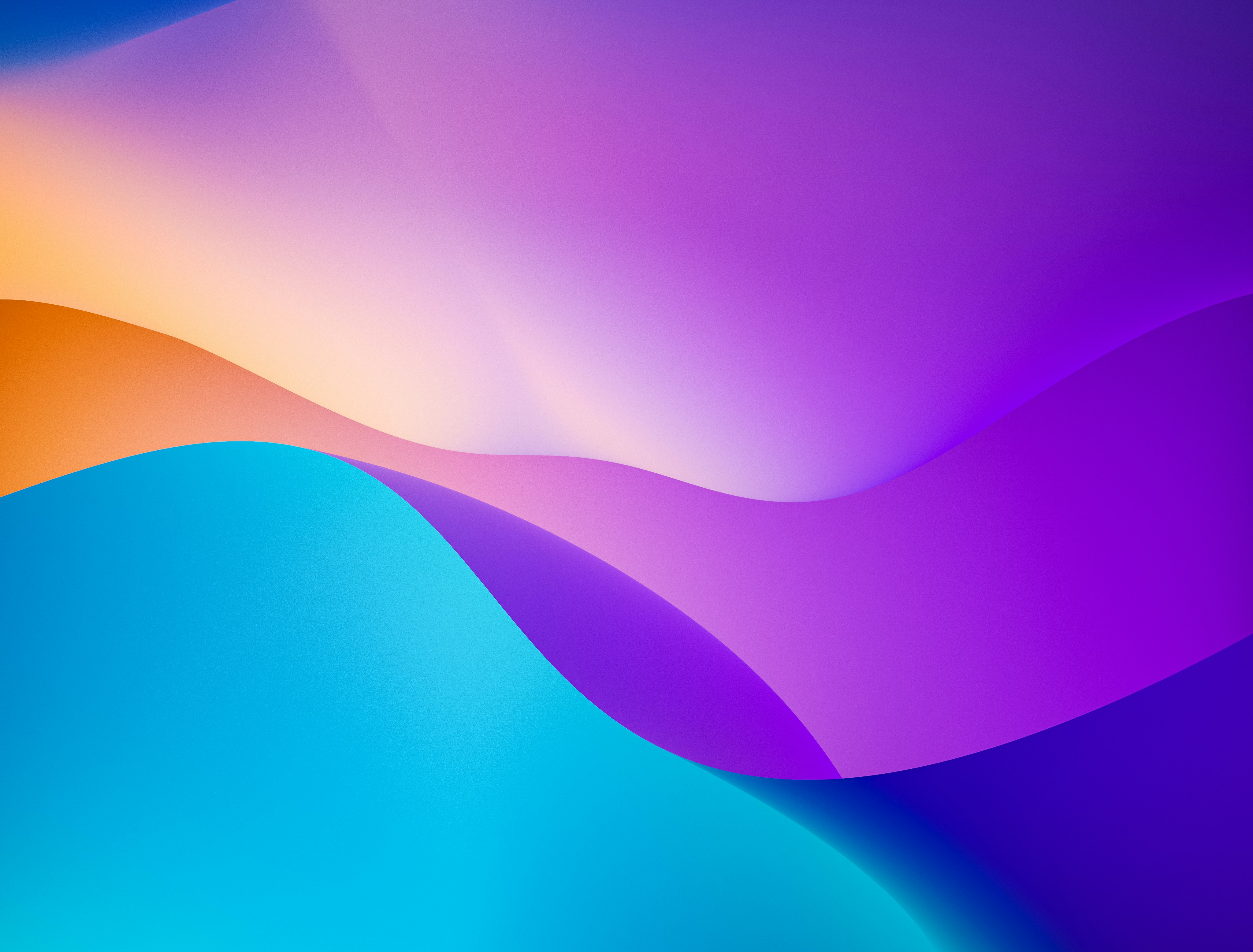
Understanding Data Quality Management
Prominent organizations recognize that data stands at the helm of driving informed decision making. It goes without saying that the quality of data funneled into these decisions is critical, this is where Data Quality Management (DQM) steps in. DQM is a clear-cut process that aims to ensure the accuracy, consistency, reliability, and overall integrity of corporate data. It’s not just about having mountain-sized heaps of data; but it's the quality that dictates the relevance and usability of this data.
Placing the spotlight on the quality of data opens up the corridors towards increased operational efficiency, risk mitigation, and conformity with regulatory standards. Moreover, superior data quality jettisons an organization's competitiveness, by enabling them to leverage accurate insights for strategic business outcomes. The key components of data quality include accuracy, integrity, consistency, completeness, and timeliness.
Ways to Measure Data Quality
Measuring data quality is broad and multi-faceted, there is no one-size-fits-all metric. Instead, diverse attributes define the quality of data.
Accuracy fundamentally portrays how close a data point comes to its true value. Ensuring accuracy is a non-negotiable aspect of DQM, as inaccurate data can mislead crucial business decisions. The absence of data errors posits as a crucial facet in deriving accurate business insights.
Integrity pertains to the consistency and trustworthiness of data over its lifecycle. It safeguards the data against alteration from its original state. Providing a secure framework that reduces data breaches, corruption, and loss, subsequently cascades into significant operational efficiency.
Consistency, in data-language, speaks of uniformity in the measures used, devoid of any discrepancies. Organizations should be able to draw parallels when comparing data sets.
Data is considered Complete when it includes all the necessary parts, information, and details it needs to serve its purpose. Incomplete data leaves space for assumptions, leading to potentially inflated or deflated metrics.
Lastly, the Timeliness aspect of data reflects on whether the data is up-to-date and available when required. Stale data could render decision-making futile, making timeliness essential to yield current and relevant business insights.
These five pillars of data quality are intrinsically linked. An impediment in one aspect can create a ripple effect, causing lapses in the overall quality of data. Therefore, as measurements of data quality, they yield a comprehensive view into the caliber of data on which businesses build their insights.
Process of Implementing Data Quality Management
Crafting an effective data quality management strategy is a multi-stage journey that involves thorough planning and continuous nurturing of processes. Here's a bird's eye view of what the process typically involves:
Data Quality Assessment - The initial stage consists of a holistic review of the existing data. Identifying current pain points, evaluating data sources, and recognizing data patterns sets the foundation for subsequent steps. The key here is to focus on business objectives and determine how data can help attain these goals.
Design and Implementation of Rules & Standards - Once baselines and objectives are defined, it becomes essential to establish the rules and standards that will guide the data quality management process. This includes defining the format and structure of data, establishing data governance policies, and setting up processes for constant data quality checks.
Data Profiling - This step involves getting to know your data intimately. Through statistical analysis and exploration of data relationships, data profiling helps in identifying anomalies, redundancies, and inconsistencies - spotlighting areas of concern in your data landscape.
Data Cleansing - Identified errors from the profiling phase then need to be rectified. Known as cleansing, this stage involves cleaning up inaccuracies, filling in missing values, removing duplicates, and standardizing data for consistency across the board. A critical component of DQM, data cleansing rejuvenates the health of your data reservoir.
Data Quality Monitoring & Improvement - The task of ensuring data quality is a continuous exercise, not a one-off event. Consistent monitoring and auditing of data quality to identify trends, detect anomalies, and verify the effectiveness of data governance is vital. Furthermore, strategies need to be continuously improved and realigned with evolving business objectives and technological advancements.
Risks of Poor Data Quality
In a world increasingly driven by big data and analytics, maintaining top-tier data quality is intimate with the stability and success of enterprises. When data quality goes south, it could usher in a myriad of risks and repercussions.
Business decision making stumbles significantly when rested on the shoulders of poor quality data. Inaccuracies or inconsistencies in data can lead to misguided decisions, adversely affecting the strategic direction of the organization.
On an operational level, low-grade data can load your processes with inefficiencies. Time and resources exploring and rectifying data issues could otherwise be channeled into core business operations.
Moreover, in industries where regulation is a critical component such as financial services or healthcare, subpar data quality can raise major compliance issues. Laws and regulations are express in their emphasis on data accuracy and integrity, and any breach can lead to severe penalties, tarnishing the company's reputation as a result.
These risks uncover the dire need for a robust Data Quality Management strategy in place. For in the absence of such a system, the seemingly benign issue of poor data quality can escalate into significant disruption. It's then, not just about data management, but intelligent data management that keeps quality in its crosshairs.
The Role of Machine Learning and AI in Data Quality Management
Unraveling the powers of Machine Learning (ML) and Artificial Intelligence (AI) in managing data quality can sharpen an organization's competitive edge in the current data-driven landscape. Their smart, automated capabilities can streamline many of the tasks involved in DQM.
For instance, Automated Data Cleansing distinguishes itself as a primary application of AI in DQM. Traditional manual data cleaning methods are becoming increasingly untenable with the explosion of big data. AI solutions step in, automatically detecting and rectifying errors, thus redefining accuracy and efficiency.
Predictive Analytics for Data Quality Improvement is another potent use of AI. By employing ML algorithms on historical data, AI can predict potential data quality issues before they occur, allowing for proactive measures. This AI-enabled foresight can help businesses mitigate the risk of data errors and maintain a consistently high standard of data quality.
Maintaining Data Consistency and Timeliness is a pressing concern for organizations handling vast data sets. AI can provide real-time data quality monitoring, ensuring that any slipped discrepancies or inconsistencies are immediately detected and amended. The use of AI ensures that the data feeding into business decisions is current and coherent.
Use Cases: Data Quality Management in Different Industries
Different industries grapple with a diverse range of data quality challenges and hence, the application of DQM varies. Let's bring to light some of these variations:
Within Financial Services, accuracy and integrity bear paramount importance as errors can lead to falsified reports, compliance issues, and severe financial losses. DQM practices help in preventing fraudulent activities, reducing risk and ensuring regulatory compliance, thereby nurturing the trust of consumers and regulators alike.
For the Healthcare sector, complete and accurate patient data is essential for efficient service delivery. Poor data quality can lead to harmful or potentially fatal mistakes in patient care. DQM helps maintain critical accuracy standards in patient databases, driving significant improvements in decision-making and patient care outcomes.
In the Governmental domain, quality data helps ensure the effectiveness of public policies and the equitable distribution of resources. With DQM, government agencies can enhance service delivery, policy-making, and track progress towards strategic goals more effectively.
The infusion of Data Quality Management into an industry's operating framework can lead to notable improvements in process effectiveness, operational efficiency, and most importantly, customer satisfaction. The deployment may vary by industry, but the underlying principle remains the same – a dedicated effort towards enhancing the pillars of data quality.
Challenges and Solutions in Implementing Data Quality Management
Navigating the path of Data Quality Management is not without its bumps. Some challenges organizations face while implementing DQM include vague understanding of data quality dimensions, lack of a unified view of data, limited staff expertise, and resistance to cultural changes.
A clear understanding of data quality dimensions and their relevance to specific business objectives is crucial to effective DQM. Implementing training programs and frequent knowledge-sharing forums can help augment the staff's understanding about the intricacies of data quality.
Breaking down data silos to achieve a unified view of data is another challenge that organizations commonly face. Leveraging state-of-the-art technologies and platforms which provide consolidated, consistent data views can be key to overcoming this barrier.
In some cases, the staff may lack the necessary expertise to manage high-quality data on a continuous basis. Here, training programs on DQM best practices, technologies, and compliance standards can prove beneficial.
Often, a change in the organization's data culture is required - from viewing data quality as a technical issue to viewing it as a business issue. Strong leadership and clear communication about the benefits of high-quality data for all business units are essential to drive such a cultural shift.
Future of Data Quality Management: A Paradigm Shift Towards Data Intelligence
The future is looking towards not just Data Quality Management, but an evolution into Data Intelligence. Organizational hierarchy is increasingly acknowledging the indispensable role data quality plays, pivoting the focus towards sophisticated data governance strategies.
This shift towards data intelligence involves an anticipatory approach rather than a reactive approach to data management. With AI and ML at the forefront, predictive and preventive data management can help organizations stay a step ahead when it comes to maintaining data quality.
More integrated, automated, and intelligent systems are predicted to dominate the data landscape, rendering traditional methods obsolete. Furthermore, enhanced data literacy across all levels of an organization will drive more accurate, data-driven decisions.
So, as we move forward, it's evident that businesses are progressing beyond just managing their data. The future is about making the data work smarter, turning data into an intelligent, strategic asset that businesses can wield for enhanced competitiveness in an increasingly data-driven world. Remember, behind every successful AI and analytics story, lies the foundation of quality data.
If you're interested in exploring how Deasie's data governance platform can help your team improve Data Governance, click here to learn more and request a demo.