Data Quality Dimension: Enhancing the Value of Your Business Data
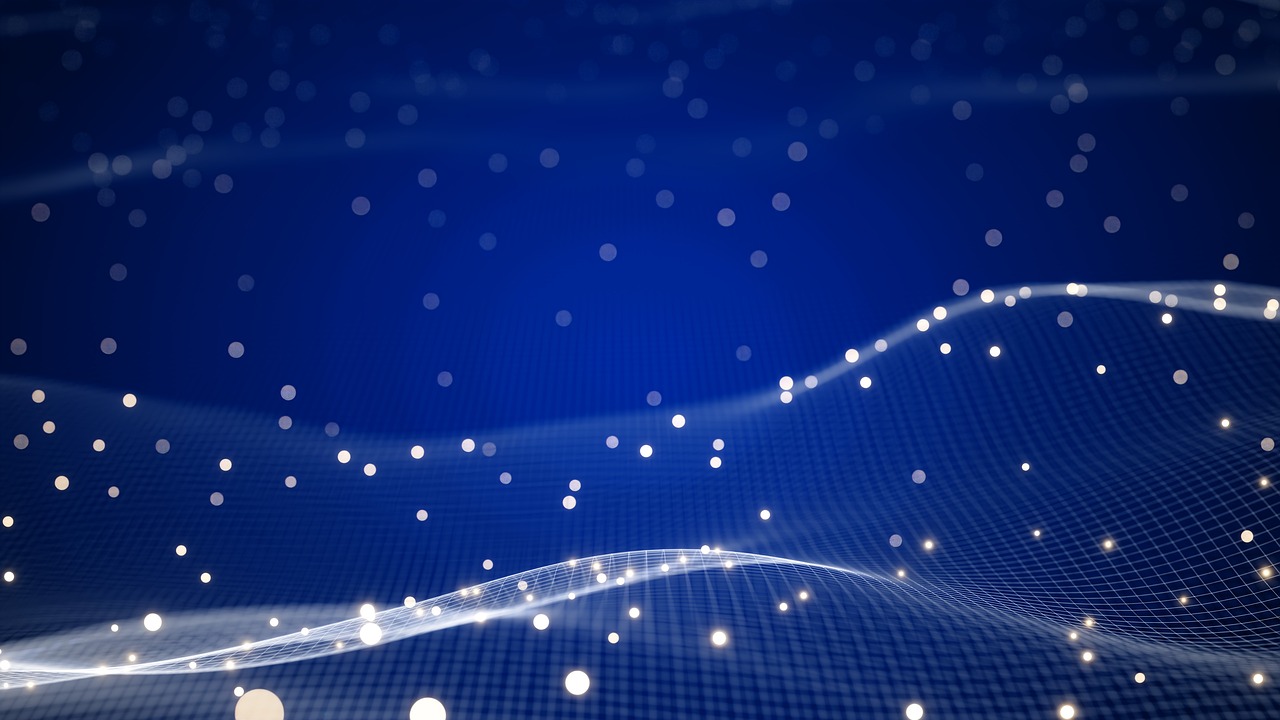
Understanding Data Quality Dimension
Let's embark on the fascinating journey of exploring data quality dimension, a concept that lies at the heart of digital transformation. Whether you're a data scientist, a business analyst, or an executive, it's imperative to understand that data quality is not just about the presence of data, but it encompasses certain dimensions that assess its fitness for use in operations, decision-making or planning.
In essence, the data quality dimension refers to the parameters or metrics used to gauge the quality of a dataset. It encapsulates several aspects — from how accurately data represents real-world variables to its consistency, completeness, uniqueness, and timeliness.
A crucial fact to absorb is that data forms the backbone of an organization's strategic, operational, and managerial decisions. But access to large volumes of data doesn't guarantee better decisions. The decisions driven by these vast data pools are as good as the quality of the data itself. Hence, beyond mere data collection, organizations must ensure their data clears the quality check across specific dimensions — the topic we'll delve into next.
Key Dimensions of Data Quality
Data quality isn't a one-dimensional concept; it radiates across multiple facets which define the integrity and usefulness of data. Let's sift through the key dimensions of data quality:
- Accuracy: This dimension gauges how well the data reflects the real-world instance it represents. Inaccurate data can lead to false insights, leading organizations astray in their strategic course.
- Consistency: Reiteration in data may sometimes deviate the expected outcome. Consistency ensures that the same data doesn't result in varying outcomes across different platforms or systems.
- Completeness: Incomplete data sets pose significant challenges in processing and decision-making. A complete data set allows for exhaustive analysis, essential for sound decisions.
- Uniqueness: One size doesn't fit all in the data world. Unique pieces of information signify distinct variables, unraveling richer, more diverse insights.
- Timeliness: Data loses its relevance over time. Therefore, a high-quality data set must be timely and relevant to the current scenario. Old, outdated data can seriously impair decision-making.
Understanding these quality dimensions will facilitate your pursuit of high-quality data, driving your business decisions closer to desired outcomes and away from projects built on unstable or faulty data foundations.
The Link Between Data Quality Dimension and Business Value
Unveiling the mystery surrounding data quality dimensions paves the way to capturing the undeniable link between data quality and business value. If data is the new oil, then data quality stands as its refinery unit, maximizing the value derived from the raw, unprocessed data.
High-quality data forms the foundation stone for making business decisions, operationalizing strategies, and shaping policies. Accuracy in data leads to accuracy in forecasting and strategy formulation. Consistent data across platforms enables integration, boosts efficiency, and ensures a single source of truth. Complete datasets render comprehensive insights, enhancing the depth and scope of business decisions. Unique data points unmask a diverse range of variables contributing to more robust analyses. Timely data helps capture the business dynamics in the here and now, enabling agile, responsive decision-making.
Further, data quality dimensions gain pronounced significance when we venture into the AI and ML arena. These technological advancements thrive on high-quality data. Faulty or low-quality data can impact the performance of AI and ML models, often leading to erroneous outputs. So, maintaining a high data quality standard becomes a prerequisite for successfully leveraging these tech advancements.
Challenges in Achieving High Data Quality
However, the path to achieving high data quality isn't devoid of obstacles. Several challenges can stand in the way, impeding the acquisition of high-quality data.
Data silos present one of the prominent challenges. The disparate, unconnected pockets of data within an organization, often known as data silos, hinder a unified view of data, leading to inconsistencies and potential inaccuracies.
The absence of a sound data governance structure is another hurdle. Lack of clear policies, improper handling of data, and the absence of standards can compromise data's reliability and consistency.
Supplementing this is the challenge of inadequate resources, both in terms of skilled personnel and powerful technological tools. The dearth of resources can dilute the effectiveness of data collection, processing, and management efforts, impacting overall data quality.
Acknowledging and addressing these challenges form an integral part of enhancing an organization's data quality and thereby, boosting the value derived from its business data. From implementing rigorous data governance strategies to deploying modern data quality tools, one needs to make concerted efforts to navigate through these challenges.
Strategies for Enhancing Data Quality
Picturing a future where high data quality is the norm requires outlining strategic steps that act as a roadmap to this end goal. Three primary strategies can pay dividends in enhancing data quality: implementing robust data governance, employing data quality tools, and ensuring continuous monitoring and maintenance.
Data governance rings the first bell. An effective data governance framework sets well-defined policies and procedures to manage an organization's data assets. It not only ensures consistency and accuracy but also enhances the reliability of data. Establishing clear ownership, creating standard definitions, setting data roles, and responsibilities are crucial facets of data governance.
Next is the role of data quality tools. These tools, packed with features for data cleaning, validation, profiling, and transformation, can conduct in-depth quality checks, identify issues, and rectify problems swiftly.
The final strategy revolves around continuous monitoring and maintenance. Data quality isn't a one-off task, but a continual process that requires regular audits, checks, and updates. This continuous approach ensures that the data remains relevant, accurate, and valuable over time.
Data Quality in Practice: A Case Study
To enrich our understanding of data quality dimensions, let's consider a hypothetical example. Suppose we have a large e-commerce business that deals with a diverse range of products from different vendors worldwide. The extensive shopping platform has enormous data related to vendors, products, sales, customers, and transactions.
Ensuring the accuracy of this data is critical - incorrect product details or inaccurate vendor information could significantly harm the customer experience and the brand's reputation. Consistency across platforms - mobile, web, or in-app, ensures a seamless user experience. The completeness of the product catalog, with exhaustive details of each product, can enhance the purchase decisions of customers. Guaranteeing the uniqueness of each product listing avoids confusion and redundancy. Timely updates of stock status, pricing, and new arrivals ensure the relevance of the data showcased to the customers.
Overcoming data quality challenges, like breaking down data silos, implementing a strong data governance mechanism, and using appropriate data quality tools, can enhance data quality.
Investing in data quality is not just an operational concern for the e-commerce platform, but it directly correlates with customer satisfaction, trust, and ultimately, the business's bottom line. This analogy brings the importance of data quality dimensions to life, reinforcing their role in effective business decision-making.
Future Trends in Data Quality Management
Change is the only constant, and this affirmation rings true even in the context of data quality management. As technology advances, data quality dimensions and management are also witnessing a remarkable transformation. The advent of AI and machine learning is reshaping how we handle data quality.
Leveraging AI, businesses can automate many data quality operations, such as data cleaning, elimination of duplicates, and correction of inconsistencies. Machine learning algorithms can learn from the data, identify patterns, and predict future data quality issues, enabling organizations to be more proactive in maintaining high data quality.
Another growing trend is the integration of data quality tools with other platforms. For instance, integrating these tools with cloud platforms or data management systems creates a more seamless and efficient means of improving data quality.
Has the future arrived? Perhaps not. But the road to it seems clear. The future of data quality management will continue to evolve with technological advancements and the growing understanding of data as a strategic asset. The journey will demand continuous learning and adaptation but promises a destination where high-quality data fuels exceptional business value.
If you're interested in exploring how Deasie's data governance platform can help your team improve Data Governance, click here to learn more and request a demo.