Data Governance: What Is It and Why It Matters for Your Business
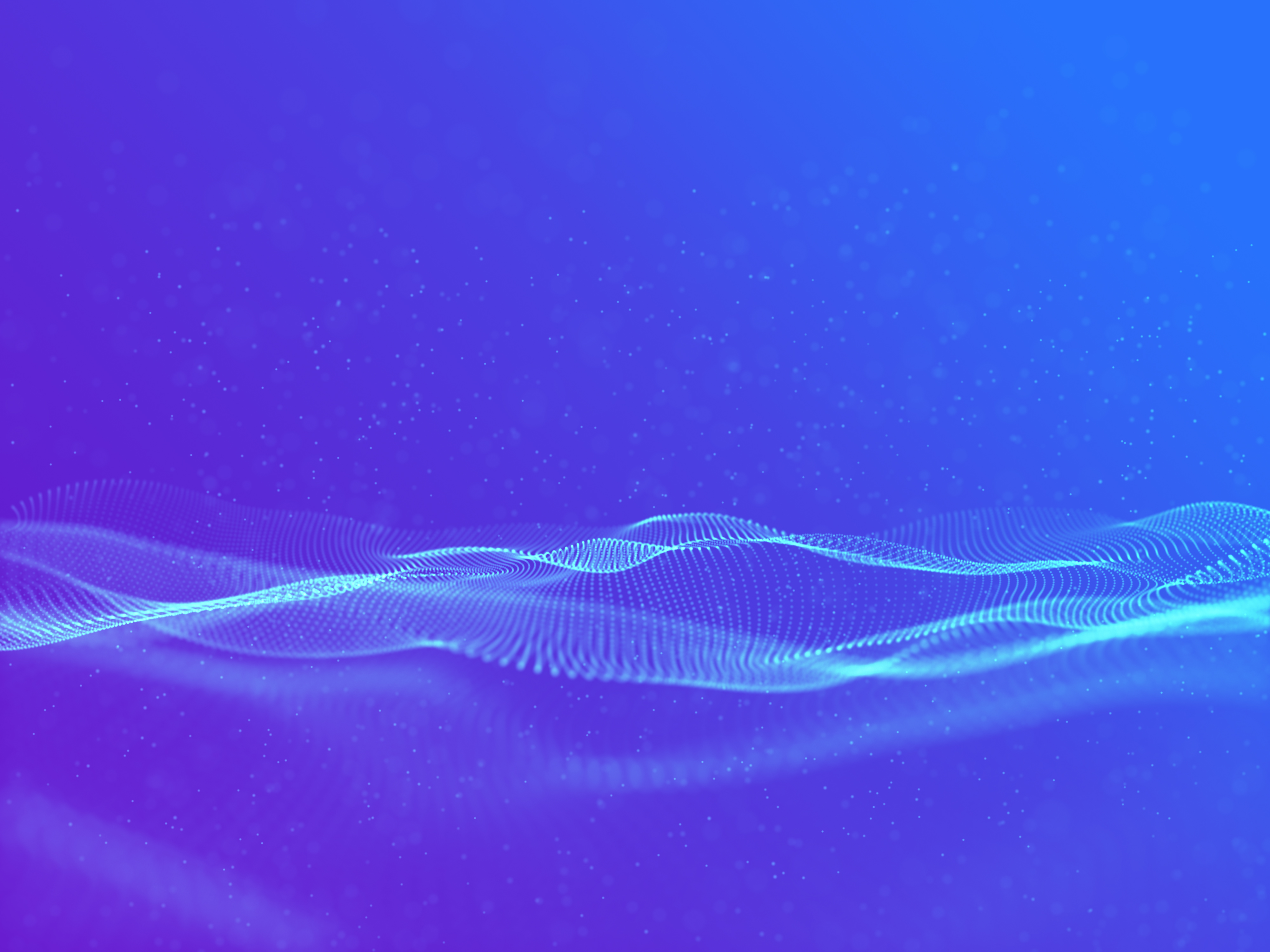
The What and Why of Data Governance
Data governance encapsulates an elaborate network of principles, practices, and procedures aimed at managing and utilizing data within an organization. It's the blueprint that guides how data should be formally managed, maintained, accessed, and stored, ensuring the underlying quality, usability, and security of this data.
Now, why should businesses care about data governance? In a business landscape powered by data, a robust data governance strategy can be a game-changer. It not only assures data quality and accuracy but also fosters regulatory compliance and risk mitigation. Furthermore, efficient data governance creates an environment wherein data can be leveraged for strategic decision-making, leading to a competitive edge.
Data governance acts as the guiding light that illuminates the path to optimized data utilization, consistency, and security. It's a cogent force that assures trust in data, forming a resilient foundation that supports all data-driven endeavors.
In the following sections, we'll dissect the core components of a data governance framework, clarify key terminologies, highlight the relationship between data governance and technology, provide implementation guidelines, and take a peek into the future trends of this dynamic discipline.
Anatomy of a Sound Data Governance Framework
A data governance framework is akin to a master blueprint. It provides a detailed view of the components integral to the successful execution of a data governance strategy.
At the very core lies the creation of Data Governance Policies. These are established guidelines and rules that stipulate how various data-related activities should be performed within the organization. From data collection and storage to usage and security, these policies give a structured path to all data operations.
Integral to the framework are Data Stewards. They are the appointed custodians of an organization's data, ensuring that it's consistently high-quality and adhering to all the policies laid down for managing the data. Acting as the bridge between data creators and users, they ensure accountability and consistency.
On an organizational level, a Data Governance Council, a team of cross-departmental members, sets the strategic direction for data governance. This council aligns the data governance objectives with broader business goals, and they monitor the overall effectiveness of the governance initiative.
Data Architecture, another crucial component, outlines the design and composition of data systems in an organization- how data is stored, transformed, integrated, and utilized. A well-designed data architecture aids in smooth data governance operations.
Taken together, these components provide a holistic, robust structure to data governance, assuring data quality, consistency, and security. In turn, this facilitates data-driven decision-making, pushing an organization closer to its business objectives.
Cutting Through the Jargon: Key Data Governance Terms
Diving deeper into data governance introduces an array of terms and definitions. Understanding these terms allows for better navigation and comprehension of data governance strategies.
Master Data Management or MDM takes the spotlight as a central concept. It denotes a coordinated set of disciplines, principles, and techniques that underscore the accuracy, uniformity, and semantic consistency of an organization's official shared master data.
Data Quality Management (DQM), as its name indicates, focuses on the practices, processes, and technologies aimed at maintaining and enhancing the quality of data. It's a key endeavour that assures the standard, completeness, and reliability of data.
In the context of data governance, Metadata Management stands for the administration and management of data that provides information about other data. In essence, it's data about data. It could range from simple details like the date of document creation, to complex details such as an item’s data lineage.
Data Privacy and Security, although broader terms, hold pivotal roles within data governance. Data Privacy refers to the acceptable use and handling of personal, private, or sensitive data. Data Security pertains to the protection of data from unauthorized access and damage throughout its lifecycle.
Lastly, Regulatory Compliance encapsulates the organization’s efforts to comply with the applicable laws and regulations. A sound data governance program acts as a trusty guide towards compliance.
The Intersection of Data Governance and Technology
No aspect of data governance remains untouched by the fingerprints of technology. From the versatile software tools that aid data governance to breakthroughs in AI and machine learning, technology has intrinsically woven itself into the fabric of data governance.
Data Governance Software Tools serve as efficient aides in the process. They facilitate automation of tasks, keep track of progress, and create easy-to-understand visualizations for better understanding. Pioneers in these tools include IBM's InfoSphere, SAS Data Governance, and Informatica Axon. These tools enrich the data governance framework with their diverse functionalities and ease of use.
Beyond tools, data governance finds a strong ally in the realms of AI and Machine Learning. Advanced machine learning algorithms have the capability to automate several aspects of data governance processes. They can aptly map data relationships and offer predictive insights for enhanced data management.
AI can significantly enhance the functionality of metadata management. With the ability to automate generation, categorization, and tagging of metadata, AI streamlines metadata management and improves accuracy.
By embedding automation and predictive capabilities, technology is the accelerant that propels data governance to elevated levels of efficiency. Integrating technology within their data governance strategy can help organizations in simplifying complex data processes, pitch for accuracy, conserve scarce resources, and notably, amplify the value that is extracted from data.
Establishing Data Governance: Guidelines for Success
Transitioning towards a robust data governance model is a harmonious blend of strategic planning and effective execution. Here are crucial practices to implement when charting the path towards successful data governance.
Kick-starting the process calls for delineating a concise Data Governance Program. This plan catering to a specific organization should be intertwined with their broader business goals and provide clear roles, responsibilities. An unambiguous roadmap with defined milestones enhances visibility and offers strategic direction.
Securing Organization-Wide Support for the data governance initiative is another crucial step. Ensuring alignment and buy-in across all levels fosters a shared understanding of the benefits and potential of good data governance. This collective commitment leads to greater success.
Data governance isn't a one-off project; rather, it's a continual cycle that demands persistent monitoring and periodic improvement. Regular audits varying in levels of complexity and detail, performance evaluations against pre-decided metrics, and continuous improvements make data governance agile and fine-tuned. Adding a feedback loop mechanism facilitates the necessary tweaks to keep the data governance program effective and aligned with changing business goals.
Incorporating these best practices reduces the complexity of initiating a data governance program. It ensures a coordinated effort towards achieving superior data quality and control.
The Road Ahead: Future of Data Governance
Navigating forward in the realm of data governance, the path unfurls promising trends and upcoming shifts. These evolving dynamics offer opportunities for organizations to enhance their data governance capabilities and derive maximum value from their data.
The amalgamation of Artificial Intelligence with metadata management is an emerging trend redefining traditional approaches. Advanced AI tools facilitate automated metadata generation, categorization, and tagging, all leading to enhanced efficiency of metadata management.
Predictive governance, another exciting frontier, applies the principles of predictive analytics to data governance. By analyzing historical patterns, future trends can be anticipated, enabling organizations to maintain their data quality proactively.
In addition, the emergence of DataOps promises to redefine how businesses handle data operations. Drawing insights from the DevOps' practices, DataOps aims to elevate the speed and accuracy of data analytics through an automated, process-oriented approach.
Moreover, with privacy and ethics becoming focal points in data discussions, data governance is being recognized for its role in ethical data handling. Ensuring data privacy and promoting responsible data use are now integral parts of modern data governance strategies.
As we delve further into the digital age, staying tuned with these trends and incorporating these innovations within current practices is a pillar of success for any data-driven business. Embracing the future of data governance is a step towards enhanced data control, improved business decisions, and sustained organizational success.
If you're interested in exploring how Deasie's data governance platform can help your team improve Data Governance, click here to learn more and request a demo.